Zulfadhli Mohamad
Senior Machine Learning Engineer at Rotor Videos- Claim this Profile
Click to upgrade to our gold package
for the full feature experience.
Topline Score
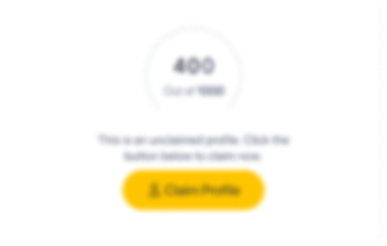
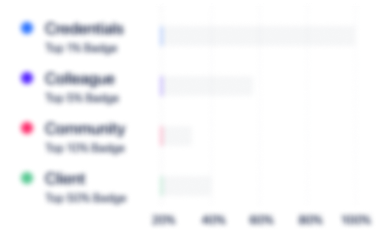
Bio


Credentials
-
Diploma of Researcher Development
Queen Mary University of LondonApr, 2018- Oct, 2024
Experience
-
Rotor Videos
-
United Kingdom
-
Media Production
-
1 - 100 Employee
-
Senior Machine Learning Engineer
-
Aug 2018 - Present
-
-
-
Queen Mary University of London
-
United Kingdom
-
Higher Education
-
700 & Above Employee
-
Researcher (PhD Student)
-
Jan 2014 - Apr 2018
• Thesis title: Estimating Performance Parameters from Electric Guitar Recordings• Motivation: Research was heavily motivated by achieving a way to copy the sound of popular guitarists from their published recordings• The best way is to extract information e.g. the type/model of electric guitar used, the settings of the instrument and guitar amps and effects used from the recordings so that users could use the extracted info in order to replicate the sound. So, machine learning techniques have been implemented to extract the info• Aim: extract relevant information from electric guitar recordings in order to replicate the sound of popular guitarists• Developed a method to morph the sound of an electric guitar pickup into another using machine learning techniques e.g least squares estimator, genetic algorithm and artificial neural network• Introduced approaches to estimate the pickup and plucking positions of an electric guitar using machine learning techniques such as linear & polynomial regression, local minima detection and clustering• Designed a technique to distinguish between electric guitar models e.g. Fender Stratocaster vs. Gibson Les Paul• Proposed a method to identify the pickup selection on an electric guitar• Performed listening tests on several participants with various musical backgrounds in order to evaluate the quality of the pickup morphing and the accuracy of the pickup and plucking positions Show less
-
-
Teaching Assistant
-
Feb 2017 - Mar 2017
Module involves analyzing and synthesizing music signals.• Teaching skills: supervised laboratory sessions for 11 master students with different scientific levels and backgrounds. Gave marks and feedbacks for students’ assignments• Communications skills: stimulated discussions with students and provided feedbacks for students to improve their lab reports
-
-
Teaching Assistant
-
Sep 2016 - Dec 2016
Modules involve modelling and analysing first and second order LTI systems in Matlab and Simulink, and designing a closed-loop control system that regulates the speed of a DC motor.• Team-work skills: facilitated laboratory sessions for 20–30 undergraduate students with 2 other Teaching Assistants, had meetings before each lab discussing methods and expected results of the labs• Leadership skills: led the group by providing answer schemes and equipment for the labs, taking students’ attendances and provided feedbacks & comments for the lecturer to improve the lab questions Show less
-
-
-
Brunel University London
-
United Kingdom
-
Higher Education
-
700 & Above Employee
-
Researcher (Master student)
-
Sep 2011 - May 2012
• Developed a mechanical pump to simulate the operation of the human heart with 2 Electrical and 2 Mechanical Engineering students • Developed a mechanical pump to simulate the operation of the human heart with 2 Electrical and 2 Mechanical Engineering students
-
-
-
-
Researcher (Undergraduate Student)
-
Sep 2010 - May 2011
• Developed and evaluated computational models of the acoustic characteristics of string musical instruments • The models are evaluated according to their capability to accurately synthesise string musical instrument signals and identify music instruments • Developed and evaluated computational models of the acoustic characteristics of string musical instruments • The models are evaluated according to their capability to accurately synthesise string musical instrument signals and identify music instruments
-
-
Education
-
Queen Mary University of London
Doctor of Philosophy (Ph.D.), Electronic Engineering -
Brunel University
Master of Engineering (MEng), Electrical and Electronics Engineering -
Universiti Kuala Lumpur
Foundation degree, Engineering
Community
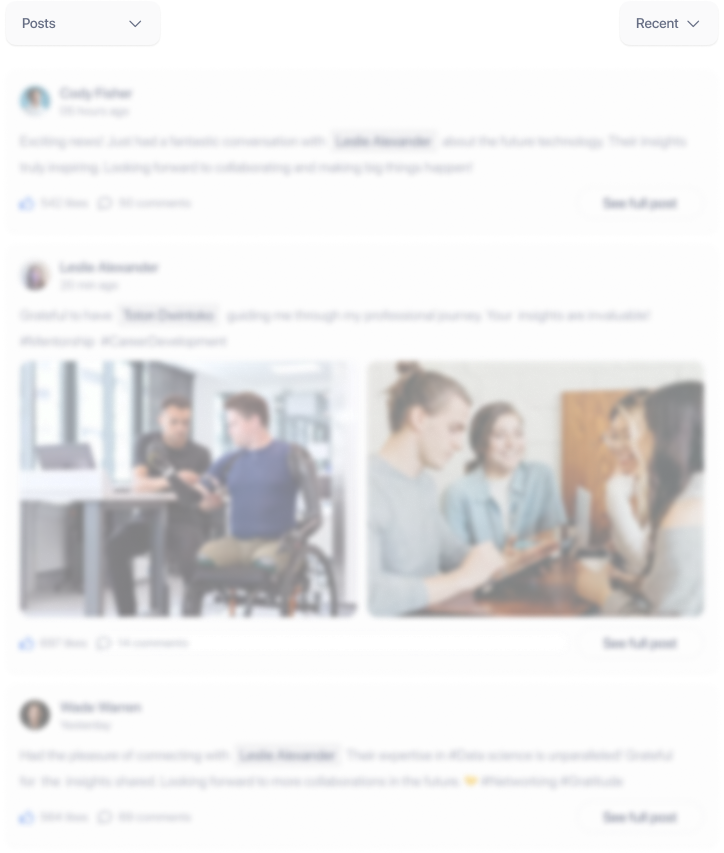