Yichuan Li
Research Engineer at HiThink- Claim this Profile
Click to upgrade to our gold package
for the full feature experience.
-
Chinese Native or bilingual proficiency
Topline Score
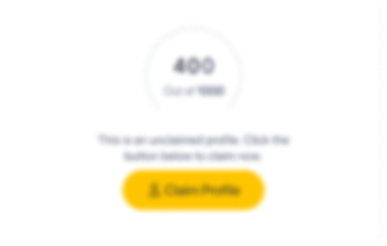
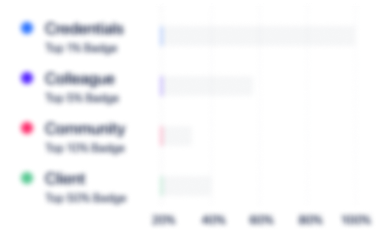
Bio
0
/5.0 / Based on 0 ratingsFilter reviews by:
Experience
-
HiThink
-
China
-
Financial Services
-
1 - 100 Employee
-
Research Engineer
-
Jul 2023 - Present
Machine learning and applications of LLM in recommendation systems. Machine learning and applications of LLM in recommendation systems.
-
-
-
University of Nevada, Reno
-
United States
-
Higher Education
-
700 & Above Employee
-
Postdoctoral Researcher
-
Dec 2022 - Jun 2023
Research on areas of optimization, control, and machine learning. Research on areas of optimization, control, and machine learning.
-
-
-
Coordinated Science Laboratory at University of Illinois
-
United States
-
Research Services
-
1 - 100 Employee
-
Research Assistant
-
May 2017 - Aug 2022
--Distributed Deep Learning (Federated Learning) Proposed a novel algorithm for Federated Learning (FedADMM). This is one of the first applications of ADMM to Federated Learning. The proposed method automatically adapts to statistical variations within data and accommodates heterogeneous network environments. Extensive numerical experiments are conducted using PyTorch on benchmark deep learning datasets (CIFAR-10, MNIST, and FMNIST), which showcase up to 87% improvement over existing… Show more --Distributed Deep Learning (Federated Learning) Proposed a novel algorithm for Federated Learning (FedADMM). This is one of the first applications of ADMM to Federated Learning. The proposed method automatically adapts to statistical variations within data and accommodates heterogeneous network environments. Extensive numerical experiments are conducted using PyTorch on benchmark deep learning datasets (CIFAR-10, MNIST, and FMNIST), which showcase up to 87% improvement over existing state-of-arts. --Decentralized Optimization (Learning) in Multi-agent Networks Proposed a general framework for designing communication-efficient curvature accelerated optimization algorithms in multi-agent settings. The proposed framework allows for a plethora of computing choices, e.g., gradient descent, Newton's method, BFGS, and L-BFGS, for agents while preserving minimal communication costs. Additional extensions for asynchronous algorithms are analyzed and implemented, which further eliminates the need for network-wide synchronization. -- Decentralized Model Predictive Control (MPC) Designed and implemented a distributed MPC algorithm for urban drainage system management. Through the use of temporal logical constraints, the proposed formulation admits efficient evaluation of mixed-integer programming along with rain forecast data. Show less --Distributed Deep Learning (Federated Learning) Proposed a novel algorithm for Federated Learning (FedADMM). This is one of the first applications of ADMM to Federated Learning. The proposed method automatically adapts to statistical variations within data and accommodates heterogeneous network environments. Extensive numerical experiments are conducted using PyTorch on benchmark deep learning datasets (CIFAR-10, MNIST, and FMNIST), which showcase up to 87% improvement over existing… Show more --Distributed Deep Learning (Federated Learning) Proposed a novel algorithm for Federated Learning (FedADMM). This is one of the first applications of ADMM to Federated Learning. The proposed method automatically adapts to statistical variations within data and accommodates heterogeneous network environments. Extensive numerical experiments are conducted using PyTorch on benchmark deep learning datasets (CIFAR-10, MNIST, and FMNIST), which showcase up to 87% improvement over existing state-of-arts. --Decentralized Optimization (Learning) in Multi-agent Networks Proposed a general framework for designing communication-efficient curvature accelerated optimization algorithms in multi-agent settings. The proposed framework allows for a plethora of computing choices, e.g., gradient descent, Newton's method, BFGS, and L-BFGS, for agents while preserving minimal communication costs. Additional extensions for asynchronous algorithms are analyzed and implemented, which further eliminates the need for network-wide synchronization. -- Decentralized Model Predictive Control (MPC) Designed and implemented a distributed MPC algorithm for urban drainage system management. Through the use of temporal logical constraints, the proposed formulation admits efficient evaluation of mixed-integer programming along with rain forecast data. Show less
-
-
-
University of Illinois at Urbana-Champaign
-
Higher Education
-
1 - 100 Employee
-
Graduate Teaching Assistant
-
2017 - 2019
Instructor of laboratory and discussion sections of ME 340 (Dynamical systems), ECE 486 (Classical Control theory), and ECE 470 (Robotics). Instructor of laboratory and discussion sections of ME 340 (Dynamical systems), ECE 486 (Classical Control theory), and ECE 470 (Robotics).
-
-
-
-
Undergraduate Research Assistant
-
Mar 2015 - Apr 2016
--Particle sorting in micro-bubble flows Conducting experiments to study the behavior of particles in microfluidic environments. --Particle sorting in micro-bubble flows Conducting experiments to study the behavior of particles in microfluidic environments.
-
-
Education
-
University of Illinois at Urbana-Champaign
Doctor of Philosophy - PhD, Optimization and Control -
University of Illinois Urbana-Champaign
Master's degree, Applied Mathematics -
University of Illinois at Urbana-Champaign
Master's degree, Mechanical Engineering -
University of Illinois at Urbana-Champaign
Bachelor’s Degree with High Honor, Mechanical Engineering
Community
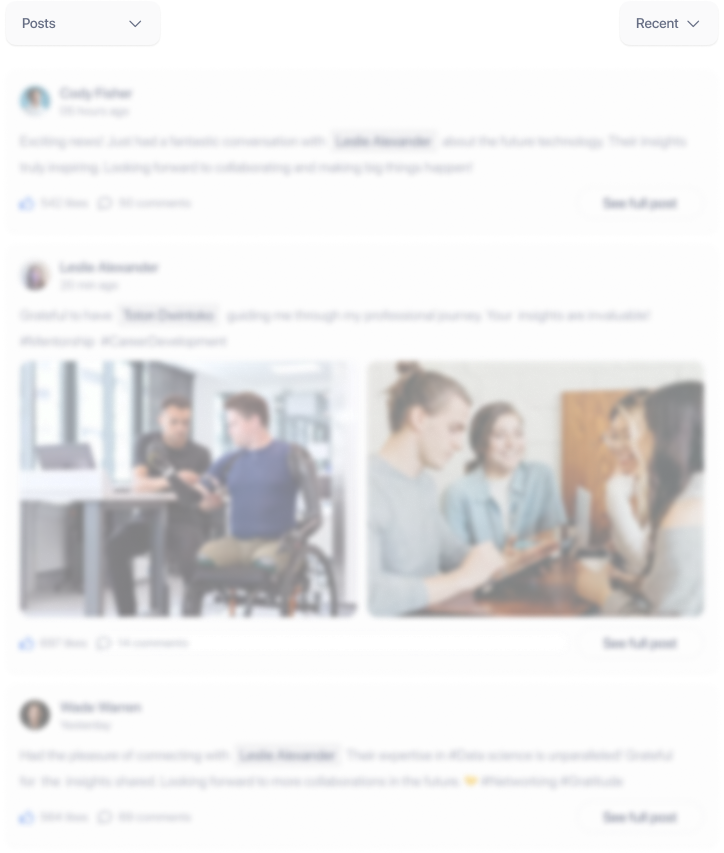