Shen Wang
Kaggle Competition Expert (Top 0.5%) at Kaggle- Claim this Profile
Click to upgrade to our gold package
for the full feature experience.
Topline Score
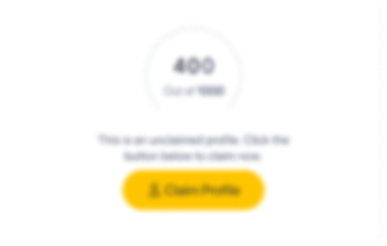
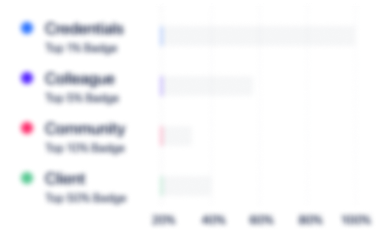
Bio


Experience
-
Kaggle
-
United States
-
IT Services and IT Consulting
-
400 - 500 Employee
-
Kaggle Competition Expert (Top 0.5%)
-
Jun 2019 - Present
Kaggle : Jane Street Market Prediction Top1% (27/4245) Kaggle : Mechanisms of Action Prediction Top1% (42/4347) Kaggle : Jane Street Market Prediction Top1% (27/4245) Kaggle : Mechanisms of Action Prediction Top1% (42/4347)
-
-
-
Kafang Technology
-
China
-
Financial Services
-
1 - 100 Employee
-
Quantitative Analyst
-
May 2022 - Sep 2022
High-frequency proprietary trading (2-ticks level) High-frequency proprietary trading (2-ticks level)
-
-
-
QTG Capital Management
-
Investment Management
-
1 - 100 Employee
-
Quantitative Research Analyst
-
Jun 2021 - Nov 2021
-
-
-
Essence Securities Co., Ltd
-
China
-
Investment Banking
-
200 - 300 Employee
-
Quantitative Analyst Assistant (Machine Learning)
-
Aug 2019 - Mar 2020
• Developed Alpha factors using China stock market abnormal signals in pre-market call auction stage. • Built a strategy backtesting framework for single factor analysis and strategy performance analysis. • Designed T+0 strategy with an average annual return of 107% and an average annual maximum callback of 9.68% • Developed Alpha factors using China stock market abnormal signals in pre-market call auction stage. • Built a strategy backtesting framework for single factor analysis and strategy performance analysis. • Designed T+0 strategy with an average annual return of 107% and an average annual maximum callback of 9.68%
-
-
-
Point Zero One Technology Ltd.
-
Hong Kong
-
Financial Services
-
Quantitative Researcher
-
May 2019 - Aug 2019
• Developed machine learning models to separate top and bottom signals of tick data from trade stocks. • Transformed 72 high correlated variables into 14 uncorrelated variables by PCA for 1 million stock transactions. Implemented and evaluated various models, including Logistic Regression, Gaussian Mixture and Quadratic Discriminant Analysis on a real-world dataset. • Implemented Semi-Supervised Learning to reinforce generalization ability, increasing win ratio from 53% to 59%. • Developed machine learning models to separate top and bottom signals of tick data from trade stocks. • Transformed 72 high correlated variables into 14 uncorrelated variables by PCA for 1 million stock transactions. Implemented and evaluated various models, including Logistic Regression, Gaussian Mixture and Quadratic Discriminant Analysis on a real-world dataset. • Implemented Semi-Supervised Learning to reinforce generalization ability, increasing win ratio from 53% to 59%.
-
-
Community
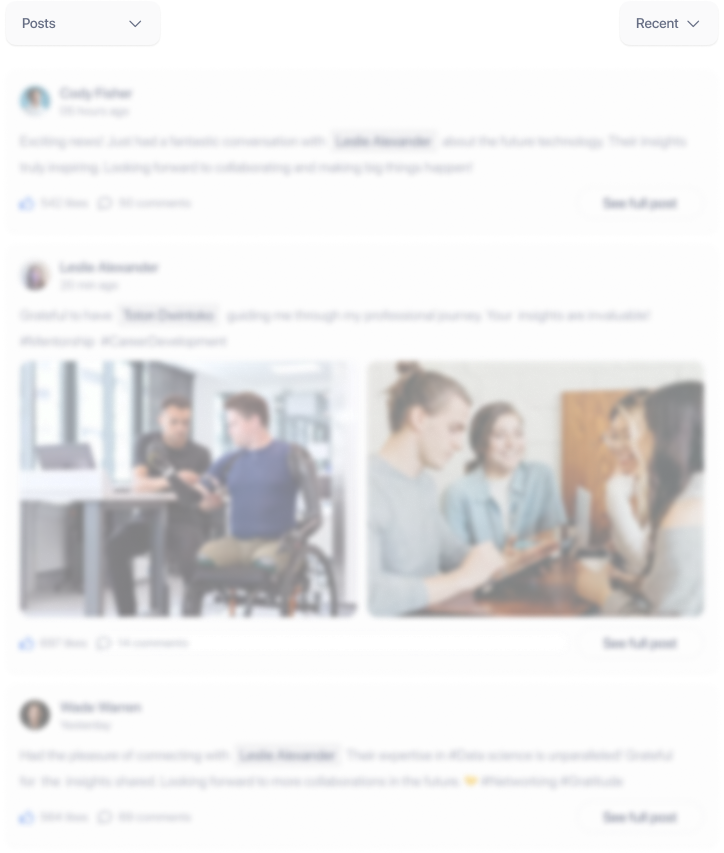