Satya Shravan
Data Scientist at Soothsayer Analytics- Claim this Profile
Click to upgrade to our gold package
for the full feature experience.
-
English Full professional proficiency
-
Hindi Full professional proficiency
-
Telugu Full professional proficiency
-
German Elementary proficiency
Topline Score
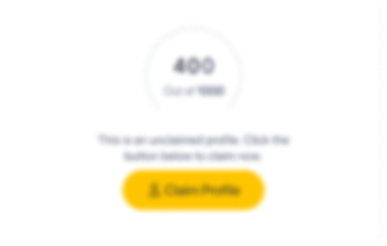
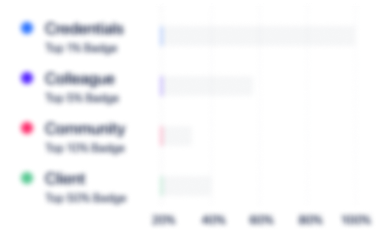
Bio

Shravan posesses exceptional technical and analytical skills and an aptitude for quickly understanding the problem statement. I had the pleasure of working with Shravan on a demanding project and his performance was flawless, delivering dependable high quality work on time. I do not hesitate endorsing Shravan for any technical position in data analytics or related field.

Shravan posesses exceptional technical and analytical skills and an aptitude for quickly understanding the problem statement. I had the pleasure of working with Shravan on a demanding project and his performance was flawless, delivering dependable high quality work on time. I do not hesitate endorsing Shravan for any technical position in data analytics or related field.

Shravan posesses exceptional technical and analytical skills and an aptitude for quickly understanding the problem statement. I had the pleasure of working with Shravan on a demanding project and his performance was flawless, delivering dependable high quality work on time. I do not hesitate endorsing Shravan for any technical position in data analytics or related field.

Shravan posesses exceptional technical and analytical skills and an aptitude for quickly understanding the problem statement. I had the pleasure of working with Shravan on a demanding project and his performance was flawless, delivering dependable high quality work on time. I do not hesitate endorsing Shravan for any technical position in data analytics or related field.

Credentials
-
Certificate in Engineering Excellence in Big Data Analytics and Optimization
Language Technologies Institute (LTI) of Carnegie Mellon University (CMU)Oct, 2015- Oct, 2024
Experience
-
Soothsayer Analytics
-
United States
-
IT Services and IT Consulting
-
1 - 100 Employee
-
Data Scientist
-
May 2017 - Present
-
-
-
AIG
-
United States
-
Insurance
-
700 & Above Employee
-
Senior Science Analyst
-
Jul 2016 - May 2017
Travel Guard Pure Premium ModellingProblem Statement: Pricing model project to predict the pure premium for customers of AIG travel insurance product, AIG Travel Guard in China and Singapore. The goal is to identify important dependent variables that determine the pricing of the product for each customer based on their past policies and claims.Responsibilities:Subset the data for each coverage code. Coverages can be of such types as Baggage delay, trip delay, Flight Cancellation, Baggage loss, theft or damage, Emergency sickness and treatment, Broken Bones, Hospital Indemnity, Loss of valuables etc.Used statistical models such as Generic linearized models, tweedie regression and regularized machine learning model Elastic net regression.Pure premium can be modelled either directly or through two variables, Frequency and Severity. Pure Premium is a product of frequency and severity.Build GLM for frequency and severity separately (since frequency is of Poisson distribution and severity is of Gamma distribution) and multiply the results to get Pure Premium.Build a tweedie regression model (Compound Poisson Gamma Model) for pure premium directly without modeling for frequency and severity.
-
-
-
Black Knight
-
United States
-
IT Services and IT Consulting
-
700 & Above Employee
-
Data Analyst
-
Jan 2015 - Jul 2016
Risk Analysis for Large Pools of LoansProblem Statement: To classify according to risk each mortgage loan within the period 1995-2014. Our data is comprised of two loan level data sets. The first is a subprime dataset consisting of over 10 million mortgages during the time period 1995-2013, obtained from the Trust Company of the West. The second data set, obtained from Freddie Mac, contains agency mortgages over the time period 1999-2014 and consists of 16 million mortgages. The mortgages are spread across the entire US, covering over 36000 different zip codes. The feature data includes zip code, FICO score, loan-to-value (LTV) ratio, initial interest rate, initial balance, type of mortgage, deal ID, and time of origination. Default, foreclosure, modification, real estate owned (REO) and prepayment events, if they occur, are also recorded in the data set on a monthly basis.Responsibilities:Data elements validation using exploratory data analysis (univariate, bivariate, multivariate analysis).Missing value treatment, outlier capping and anomalies treatment using statistical methods.Dummy variables were created for certain datasets to fit into the regression. Variable selection was done by performing forward stepwise regression, R-square and VIF values.Fit a logistic regression model using the entire subprime data set prior to January 1, 2012.The loan-level features used for both default and prepayment are FICO score, LTV, initial balance of the mortgage, and initial interest rate for the mortgage.
-
-
-
LTI - Larsen & Toubro Infotech
-
India
-
IT Services and IT Consulting
-
700 & Above Employee
-
Data Analyst
-
Oct 2013 - Dec 2014
Project: Predicting Customer ChurnProblem Statement: To predict the attrition in personal insurance products. The Churn prediction model predicts a customer's propensity to churn by using information about the customer such as household and financial data, transactional data, and behavioral data. The inputs for the Churn prediction model are customer demographic data, insurance policies, premiums, tenure, claims, complaints, and the sentiment score from past surveys.Responsibilities:Aggregate all available information about the customer. The data that is obtained for predicting the churn is classified in the following categories:Demographic data, such as age, gender, education, marital status, employment status, income, home ownership status, and retirement plan.Policy-related data, such as insurance lines, number of policies in the household, household tenure, premium, disposable income, and insured cars.Claims, such as claim settlement duration, number of claims that are filed and denied.Complaints, such as number of open and closed complaints.Survey sentiment data. Sentiment scores from past surveys are captured in the latest and average note attitude score fields. The note attitude score is derived from customer negative feedback only. If the note attitude is zero, the customer is more satisfied while as the number increases, satisfaction level decreases.Responsible for building data analysis infrastructure to collect, analyze, and visualize data.Data elements validation using exploratory data analysis (univariate, bivariate, multivariate analysis).Missing value treatment, outlier capping and anomalies treatment using statistical methods.Variable selection was done by making use of R-square and VIF values.Deployed Machine Learning (Logistic Regression and PCA) to predict customer churn.
-
-
-
Capgemini
-
France
-
IT Services and IT Consulting
-
700 & Above Employee
-
Associate Consultant
-
Jul 2010 - May 2013
Project: One HSBCResponsibilities:Worked on different tasks of data analytics pipeline like data cleaning/transformation, and exploratory analysis of data sets using R and SQL.Responsible for the design and development of Business Intelligence (BI) reports and dashboards for data visualization.Responsible for communicating functional requirements to the entire team and training new colleagues of the project.
-
-
-
Infosys
-
India
-
IT Services and IT Consulting
-
700 & Above Employee
-
Senior Systems Engineer
-
May 2007 - Jan 2010
Roles and Responsibilities:Responsible to write stored procedures, triggers, views etc., in MS SQL Server.Responsible for performance tuningDevelop components of databases, data schema, data storage, data queries, data transformations, and data warehousing applications.Analyzing Problems in the system and making recommendations for system improvement.Developing custom reporting solutions using SQL Server.Actively participate in group technology reviews to critique work of self and others.
-
-
Education
-
International School of Engineering
Certificate Program in Engineering Excellence, Big Data Analytics and Optimization -
GITAM college of Engineering, Andhra University
Bachelor of Engineering, Mechanical Engineering
Community
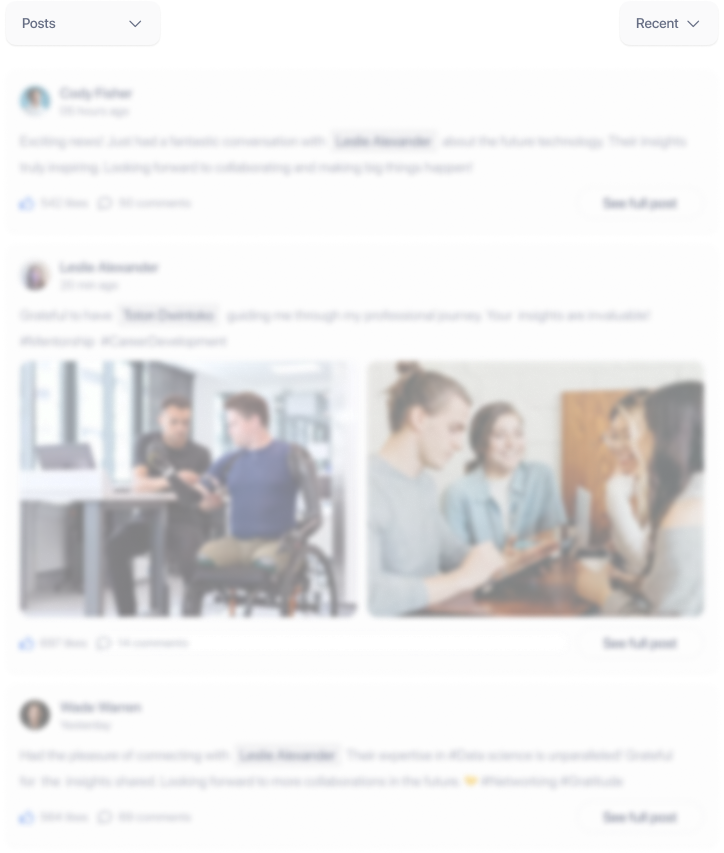