
Mihir Patil
Computer Vision Engineer at rabbitAI- Claim this Profile
Click to upgrade to our gold package
for the full feature experience.
-
German Elementary proficiency
-
English Native or bilingual proficiency
-
Marathi Native or bilingual proficiency
-
Hindi Native or bilingual proficiency
-
Gujarati Full professional proficiency
Topline Score
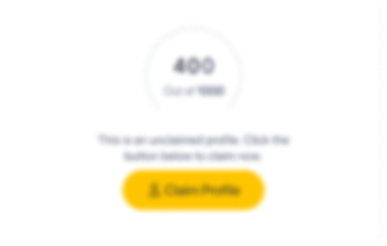
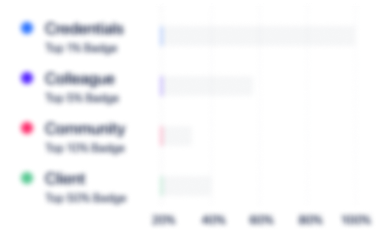
Bio


Credentials
-
Introduction to Machine learning in Production
CourseraJul, 2021- Nov, 2024 -
Sequence Models
CourseraDec, 2019- Nov, 2024 -
Deep Learning Specialization
CourseraDec, 2019- Nov, 2024 -
Neural Networks and Deep Learning
CourseraNov, 2019- Nov, 2024 -
Introduction to Self-Driving Cars
CourseraNov, 2019- Nov, 2024 -
Improving Deep Neural Networks: Hyperparameter tuning, Regularization and Optimization
CourseraOct, 2019- Nov, 2024 -
Structuring Machine Learning Projects
CourseraOct, 2019- Nov, 2024 -
Machine Learning
CourseraAug, 2019- Nov, 2024 -
Robotics: Estimation and Learning
CourseraJul, 2019- Nov, 2024
Experience
-
rabbitAI
-
Germany
-
Information Technology & Services
-
1 - 100 Employee
-
Computer Vision Engineer
-
Sep 2021 - Present
-
-
-
Deutsches Forschungszentrum für Künstliche Intelligenz (DFKI)
-
Germany
-
Research Services
-
700 & Above Employee
-
Student Research Assistant
-
Jun 2020 - Apr 2021
• Building a custom deep reinforcement learning environment for underwater docking of the Deepleng AUV using the OpenAI-Gym framework.• Implementing deep reinforcement learning agents based on state-of-the-art algorithms such as SAC, PPO, TD3, DDPG.• Conceptualization and deployment of an end-to-end deep reinforcement learning pipeline to train and test learning agents.
-
-
Master Thesis Project - Deep Reinforcement Learning for Continuous Control Docking of AUVs
-
Jul 2020 - Jan 2021
Docking control of an autonomous underwater vehicle (AUV) is a task, integral to achieving persistent long term autonomy in the field of underwater robotics. The work done in this thesis therefore explored the application of state-of-the-art model-free deep reinforcement learning (DRL) approaches to the task of 2-dimensional underwater docking in the continuous domain. While using a state-space defined by the pose, velocity and thruster values of the AUV. With the goal of learning real-valued thruster outputs required to control the AUV. It detailed a step by step formulation of the reward function that was used to successfully dock the AUV onto a fixed docking platform. A major contribution that distinguishes this work from the previous approaches is the usage of a physics simulator to define and simulate the underwater environment as well as the DeepLeng AUV. Moreover, this work evaluated state-of-the-art DRL algorithms such as proximal policy optimization (PPO), twin delayed deep deterministic policy gradients (TD3) and soft actor-critic (SAC) in combination with the developed reward function, using metrics such as the average episodic rewards, average steps required to reach the docking station and the success rate. This empirical evaluation yielded results that conclusively show the TD3 agent to be the most efficient and consistent in terms of docking the AUV, over multiple evaluation runs it achieved a 100% success rate with an average trajectory length of 31 steps and an average episodic return of 10667.1 +/- 688.8. Through comparative evaluation amongst various reward functions, we were also able to demonstrate how the developed reward function improves upon the current state-of-the-art approach in terms of the behavior learned by the agent and thereby producing shorter and quicker trajectories to the goal, when starting from any position within 9 meters of the docking station .
-
-
-
AGT International
-
Technology, Information and Internet
-
1 - 100 Employee
-
Computer Vision / Data Science Intern
-
Jan 2020 - Mar 2020
• Establishing the data collection and transformation pipeline for training 2-D pose estimation networks. • Code migration from Tensorflow and Pytorch to Pytorch Lightning and extension of the 2-D pose estimation pipeline for 3-D pose estimation.• Engineering, training, validation of Pytorch based Neural Network for 3-D human pose estimation and direction estimation.• Hyperparameter optimization and validation of multiple 3-D pose estimation, deep learning models for direction estimation.
-
-
-
Fraunhofer FKIE
-
Germany
-
Research
-
100 - 200 Employee
-
Student Research Assistant
-
Jan 2019 - Jan 2020
• Data cleaning and visualization of raw multi-array sonar data for shooter localization in the AutoAugur project.• Dataset generation of spectrograms from sonar data to allow for classification and detection of different gunshots and types using CNN models.• Optimization and fine-tuning deep learning models to improve classification accuracy by 15%.• Development of a pipeline to localize the position(global coordinates) of a shooter, based on detected gunshots. • Data cleaning and visualization of raw multi-array sonar data for shooter localization in the AutoAugur project.• Dataset generation of spectrograms from sonar data to allow for classification and detection of different gunshots and types using CNN models.• Optimization and fine-tuning deep learning models to improve classification accuracy by 15%.• Development of a pipeline to localize the position(global coordinates) of a shooter, based on detected gunshots.
-
-
Education
-
Bonn-Rhein-Sieg University of Applied Sciences
Master of Science - MS, Autonomous Systems -
B V B College of Engg. & Technology, HUBLI
Bachelor's degree, Automation and Robotics
Community
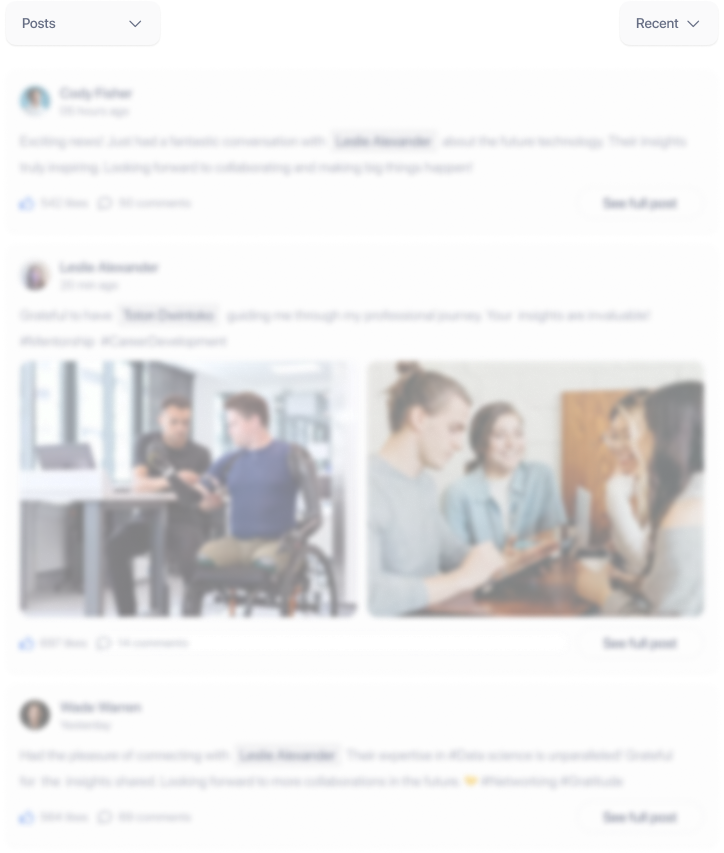