Manjeera Jagiri
Associate Lead, Data Science at Flutura Decision Sciences & Analytics- Claim this Profile
Click to upgrade to our gold package
for the full feature experience.
-
English Native or bilingual proficiency
-
Hindi Professional working proficiency
-
French Elementary proficiency
Topline Score
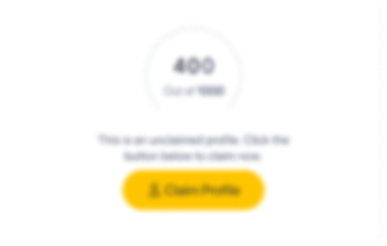
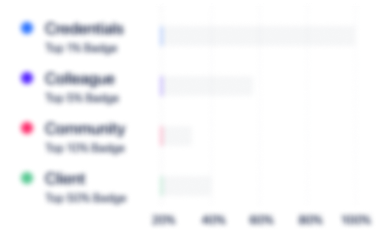
Bio


Credentials
-
SQL for Data Science
CourseraJun, 2023- Nov, 2024 -
Numero Uno
Flutura Decision Sciences & AnalyticsJul, 2019- Nov, 2024 -
A1 Level
DELF (Diploma in French Studies) -
NCFM : Financial Markets
National Stock Exchange of India Limited
Experience
-
Flutura Decision Sciences & Analytics
-
United States
-
Software Development
-
100 - 200 Employee
-
Associate Lead, Data Science
-
Apr 2021 - Present
Performance Monitoring Application, reducing unplanned downtime by monitoring asset health• Drove the interaction and partnership between engineering and data science teams to ensure active cooperation in identifying as well as defining analytical needs, and generating the pull-through of technical insights • Collaborated with PMs for project planning, development and execution encompassing the data science life cycle. Reviewed hypotheses and finalized model approaches balancing accuracy with simplicity, stability and business interpretability• Actively engaged clients to evaluate statistical and analytical models’ findings, and business users for continuous iteration and productive dialogues on what is pertinent and actionable for them• Predicted anomalous operating conditions, indicative of developing fault, in compressors to generate a prioritized alert system• Extracted steady-state operational behaviour using Gaussian Mixture Model and Bayesian Information Criterion for model selection. Reduced the susceptibility of prognostic technique by minimizing the probability of false alarms• Characterized the operating envelope into meaningful clusters using K-means clustering. Identified anomalies by calculating cluster outlier scores based on ED and CMGOS. Facilitated modeling process by constructing temporal features, which included lag, window and STL features• Analysed similarity–based modeling (SBM) techniques to model battery degradation in vehicles. Evaluated prediction errors based on Gaussian distribution to find the probability of abnormal behaviour• Compiled and analyzed vehicular sensor data across user profiles to identify and validate context-aware driving behaviour patterns, and reduce bias in calculation of driving scores Show less
-
-
Senior Programmer, Data Science
-
Apr 2019 - Apr 2021
Gas Gathering System Application, developing a simulation environment for off-field analysis• Automated detection of anomalous trend shifts in flow and pressure across the network using bayesian change point analysis• Modelled network behaviour with LSTM neural networks to predict flow, pressure and temperature distributions at critical intersections and sales points. Achieved <10% deviation on simulating varying operating ranges and shutdowns in the network• Customized the loss function of the model to penalize predictions invalidated by gas flow principles in pipeline systems• Estimated confidence scores of predictions using OpenMax method, based on probability distribution of activation vectors• “Artificial Intelligence Based Optimization of Gathering System”, ADIPEC, Abu Dhabi, UAE Show less
-
-
Programmer, Data Science
-
Jul 2017 - Apr 2019
Process Optimization Applications, improving yield quality in continuous manufacturing plants• Provided key interpretable business insights on process limits powered by decision trees and custom algorithms • Built tree based (Random forest and XGBoost) and hierarchical clustering models on domain and statistically engineered features to predict quality grade of catalysts• Extended the model framework to an automated pipeline for scalability and on-the-fly continuous model training and deployment• Developed a prediction and simulation pipeline for continuous processes using historic and knn imputed real-time data • Conceptualized and implemented a set point optimization framework for ongoing processes in a constrained environment. Recommended future values of controllable variables, using Bayesian optimization and Genetic algorithm, to maximize quality• Created an internal tool to automate data quality assessment and generate reports based on customized statistical metricsVideo Analytics Application, leveraging deep learning technology for intelligent surveillance • Built and trained object localization modules(Yolo and SSD) as anomaly event indicators for use cases(Personal Protective Equipment’s detection) in Oil and Gas plant environments. • Enhanced model credibility by using PCA and t-SNE for dimensionality reduction and visualization of images’ feature vectors• Deployed the solution with an alert system on a gateway device integrated with a CCTV for Edge Video Analytics • Created an internal tool that sets up Amazon Mechanical Turk’s Human Intelligence Task template for labelling images, and also retrieving and formatting the images’ bounding box annotationsCo-conducted a meetup session on ‘Best Practices in Time Series Analysis’ as the speaker Show less
-
-
-
SLB
-
United States
-
Technology, Information and Internet
-
700 & Above Employee
-
Summer Intern - Field Engineer | Process Optimization
-
May 2016 - Jun 2016
• Analysed workflows via bottleneck analysis • Improved process visualizations • Analysed workflows via bottleneck analysis • Improved process visualizations
-
-
-
Titan Company Limited
-
India
-
Retail
-
700 & Above Employee
-
Internship | Quality Control Management
-
May 2015 - Jul 2015
• Optimized volume of adhesives’ and UV curing period for 900 sample watch cases (9 types of watch models) • Reduced rejection rate by 15% by adopting adhesive quantization formula based on its shear and tensile strength • Predicted adhesive flow behavior with ~92% accuracy by correlating training data and fluid dynamic analysis • Optimized volume of adhesives’ and UV curing period for 900 sample watch cases (9 types of watch models) • Reduced rejection rate by 15% by adopting adhesive quantization formula based on its shear and tensile strength • Predicted adhesive flow behavior with ~92% accuracy by correlating training data and fluid dynamic analysis
-
-
Education
-
Indian Institute of Technology, Madras
Master of Technology - MTech, Mechanical Engineering -
Indian Institute of Technology, Madras
Bachelor of Technology - BTech, Mechanical Engineering
Community
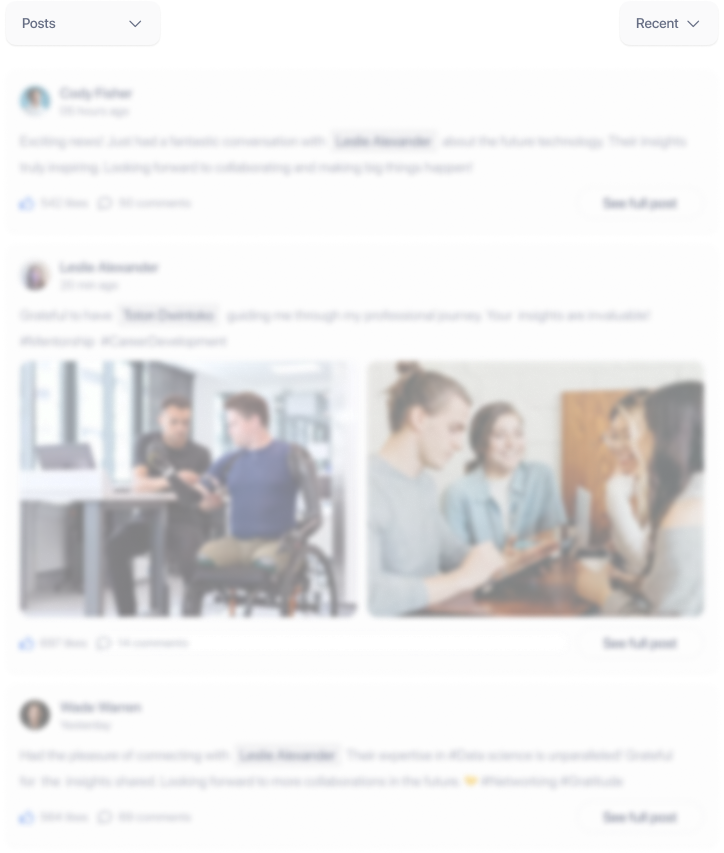