KIN YAN HO
AI GAN model trainer (Image Restoration Analysis with Deep Learning model Optimization) at Caritas Institute of Higher Education- Claim this Profile
Click to upgrade to our gold package
for the full feature experience.
Topline Score
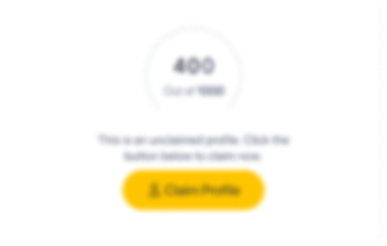
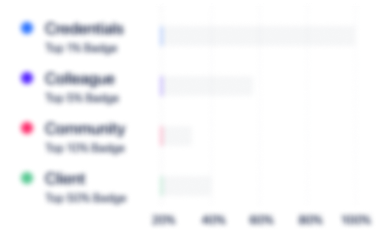
Bio


Experience
-
Caritas Institute of Higher Education
-
Hong Kong
-
Higher Education
-
1 - 100 Employee
-
AI GAN model trainer (Image Restoration Analysis with Deep Learning model Optimization)
-
Dec 2022 - Present
To apply AI (Deep learning) as a tool for the restoration and preservation of damaged images and to reveal its original contents To search/capture/collect dataset of the damaged and undamaged image pairs for training the deep learning models To generate dataset of original/undamaged/damaged image pair for training of deep learning optimization Capture pictures of undamaged object and search the image for their corresponding damaged objects To explore the capability of deep learning in the restoration of damaged images using the Egan To investigate the enhanced Generative Adversarial Networks (eGAN) to restore damaged images involving major structural damage such as scratches, bending, compression or strain Show less
-
-
-
Gaming Company
-
Computer Games
-
1 - 100 Employee
-
AI GAN model specialists (Collaborative project between laboratory and gaming company)
-
Jan 2021 - Present
Data collection: The face in the game engine includes various dimensions such as eye size, width, angle, etc., and each dimension can be controlled by a numerical value. In order to obtain the matching data between the parameter value and the game character's face photo, the gaming company provided a script based on Unity3D, which can adjust the parameter size arbitrarily and obtain the face rendering of the game character, and finally take a screenshot of the front of the game character's face. This way, the matching data between parameters and game character face photos can be obtained Use the obtained game character matching data to train our GAN model, so that random initialization parameters can generate game character face photos Randomly initialize the parameters, generate a random game character face photo, and compare it with the real player face photo uploaded by the user. The comparison is to segment and recognize the faces of the game character and the real person, calculate the similarity and contour similarity of the two, and obtain the segmentation loss and recognition loss through weighting to get the final loss. Finally, update the initial initialization parameters through gradient backpropagation. After 20 iterations, stop training and keep the final parameters Import the final retained parameters into the game engine to get the game character's face photo Show less
-
-
-
Bigo Live Vietnam
-
Vietnam
-
Technology, Information and Internet
-
1 - 100 Employee
-
IT VOICE LABELING PROJECT SPECIALIST
-
Jun 2019 - 2020
Listening to Cantonese and English daily dialogues to identify the audio status and dismiss the broken content, generating character and sound classification. Operating the voice labeling platform using IT skills and knowledge. Listening to Cantonese and English daily dialogues to identify the audio status and dismiss the broken content, generating character and sound classification. Operating the voice labeling platform using IT skills and knowledge.
-
-
Education
-
Caritas Institute of Higher Education
BSc in Artificial Intelligence, Artificial Intelligence -
Hong Kong Institute of Technology
Associate Degree of Arts, Crime and Investigation
Community
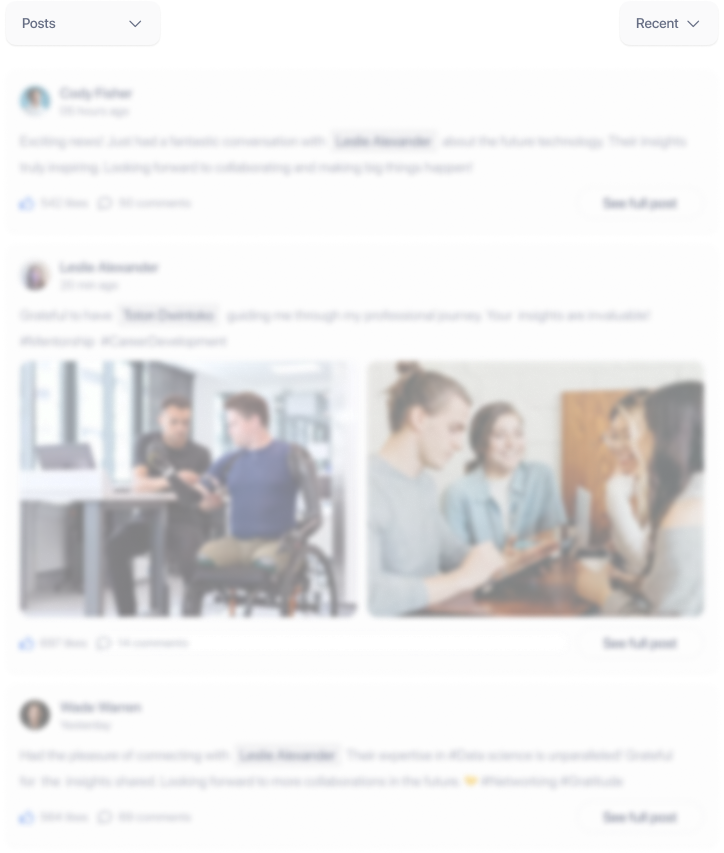