Kim Falk
Principal Data Scientist, Discovery and Recommendations at ViSenze- Claim this Profile
Click to upgrade to our gold package
for the full feature experience.
-
English Native or bilingual proficiency
-
Danish Native or bilingual proficiency
-
Italian Full professional proficiency
Topline Score
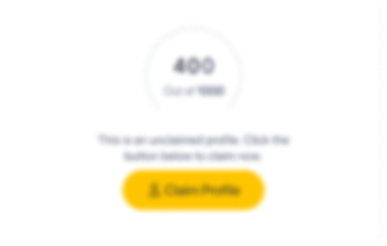
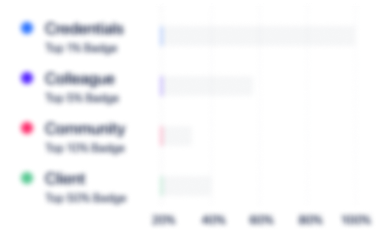
Bio

Caroline Rioux
I had the pleasure to work with Kim for 16 months at Shopify. I sought Kim out directly as I wanted to renew our investment in product recommendations, and wanted to build a new team around him. I was delighted he took on the challenge. During his time on our team Kim helped expand our API to round out of recommendations offering, oversaw the release of new ML models, helped onboard many new ML engineers and data scientists and contributed greatly to our offline and online evaluation approach, including working on personalization. Kim contributes at all levels: as a technical mentor, model developer, but where he made the biggest contribution was setting our North Star direction, under which we guided all smaller projects. A leader in the field, Kim is still approachable and thoughtful and most importantly cares deeply about the team and contributing to work that matters! I would happily work with Kim again.

Doug Turnbull
Kim is a thoughtful, brilliant recommendations systems and machine learning expert. He helped advocate for best practices around testing and evaluating recsys, part of the team that led to double-digit% GMV growth for merchants on that surface. More importantly he's a fantastic human being. He cares deeply for his colleagues, and hopes to develop them into great engineers and data scientists in the recsys space. I treasure my long conversations with Kim - we worked closely and collaborated at Shopify. I was the staff eng on search side, and him on the recsys side. So we had a lot of long conversations comparing / contrasting search and recsys. But always with shared principals of solid methodological backing to experimentation before all else Any team would be lucky to have Kim

Caroline Rioux
I had the pleasure to work with Kim for 16 months at Shopify. I sought Kim out directly as I wanted to renew our investment in product recommendations, and wanted to build a new team around him. I was delighted he took on the challenge. During his time on our team Kim helped expand our API to round out of recommendations offering, oversaw the release of new ML models, helped onboard many new ML engineers and data scientists and contributed greatly to our offline and online evaluation approach, including working on personalization. Kim contributes at all levels: as a technical mentor, model developer, but where he made the biggest contribution was setting our North Star direction, under which we guided all smaller projects. A leader in the field, Kim is still approachable and thoughtful and most importantly cares deeply about the team and contributing to work that matters! I would happily work with Kim again.

Doug Turnbull
Kim is a thoughtful, brilliant recommendations systems and machine learning expert. He helped advocate for best practices around testing and evaluating recsys, part of the team that led to double-digit% GMV growth for merchants on that surface. More importantly he's a fantastic human being. He cares deeply for his colleagues, and hopes to develop them into great engineers and data scientists in the recsys space. I treasure my long conversations with Kim - we worked closely and collaborated at Shopify. I was the staff eng on search side, and him on the recsys side. So we had a lot of long conversations comparing / contrasting search and recsys. But always with shared principals of solid methodological backing to experimentation before all else Any team would be lucky to have Kim

Caroline Rioux
I had the pleasure to work with Kim for 16 months at Shopify. I sought Kim out directly as I wanted to renew our investment in product recommendations, and wanted to build a new team around him. I was delighted he took on the challenge. During his time on our team Kim helped expand our API to round out of recommendations offering, oversaw the release of new ML models, helped onboard many new ML engineers and data scientists and contributed greatly to our offline and online evaluation approach, including working on personalization. Kim contributes at all levels: as a technical mentor, model developer, but where he made the biggest contribution was setting our North Star direction, under which we guided all smaller projects. A leader in the field, Kim is still approachable and thoughtful and most importantly cares deeply about the team and contributing to work that matters! I would happily work with Kim again.

Doug Turnbull
Kim is a thoughtful, brilliant recommendations systems and machine learning expert. He helped advocate for best practices around testing and evaluating recsys, part of the team that led to double-digit% GMV growth for merchants on that surface. More importantly he's a fantastic human being. He cares deeply for his colleagues, and hopes to develop them into great engineers and data scientists in the recsys space. I treasure my long conversations with Kim - we worked closely and collaborated at Shopify. I was the staff eng on search side, and him on the recsys side. So we had a lot of long conversations comparing / contrasting search and recsys. But always with shared principals of solid methodological backing to experimentation before all else Any team would be lucky to have Kim

Caroline Rioux
I had the pleasure to work with Kim for 16 months at Shopify. I sought Kim out directly as I wanted to renew our investment in product recommendations, and wanted to build a new team around him. I was delighted he took on the challenge. During his time on our team Kim helped expand our API to round out of recommendations offering, oversaw the release of new ML models, helped onboard many new ML engineers and data scientists and contributed greatly to our offline and online evaluation approach, including working on personalization. Kim contributes at all levels: as a technical mentor, model developer, but where he made the biggest contribution was setting our North Star direction, under which we guided all smaller projects. A leader in the field, Kim is still approachable and thoughtful and most importantly cares deeply about the team and contributing to work that matters! I would happily work with Kim again.

Doug Turnbull
Kim is a thoughtful, brilliant recommendations systems and machine learning expert. He helped advocate for best practices around testing and evaluating recsys, part of the team that led to double-digit% GMV growth for merchants on that surface. More importantly he's a fantastic human being. He cares deeply for his colleagues, and hopes to develop them into great engineers and data scientists in the recsys space. I treasure my long conversations with Kim - we worked closely and collaborated at Shopify. I was the staff eng on search side, and him on the recsys side. So we had a lot of long conversations comparing / contrasting search and recsys. But always with shared principals of solid methodological backing to experimentation before all else Any team would be lucky to have Kim

Credentials
-
Personalized Recommendations Certificate
Uplimit (Formerly CoRise)Oct, 2023- Oct, 2024 -
Practical A/B Testing
CoRiseJun, 2023- Oct, 2024 -
ml powered search
SphereNov, 2022- Oct, 2024 -
Fundamentals of Reinforcement Learning
CourseraAug, 2019- Oct, 2024 -
Introduction to Apache Spark and AWS
CourseraAug, 2018- Oct, 2024 -
Deep Learning Specialization
CourseraMay, 2018- Oct, 2024 -
Mining Massive Datasets
CourseraDec, 2014- Oct, 2024 -
Data Analysis with R
UdacitySep, 2014- Oct, 2024 -
Introduction to Recommender Systems
CourseraDec, 2013- Oct, 2024 -
Introduction to Data Science
CourseraJun, 2013- Oct, 2024 -
Web Intelligence and Big Data
CourseraJun, 2013- Oct, 2024 -
MongoDB for Java Developers
MongoDB, Inc.Apr, 2013- Oct, 2024 -
Machine Learning
CourseraOct, 2012- Oct, 2024 -
Website .NET Developer Certification for Sitecore CMS 6.5
SitecoreOct, 2012- Oct, 2024 -
Microsoft Certified Professional(MCP): ASP.NET, .NET 4.0
-Jan, 2010- Oct, 2024 -
Microsoft Certified Professional(MCP): Application Development Foundation, .NET 3.5
-Jan, 2009- Oct, 2024 -
IBM Rational Essentials of RUP
-Jan, 2004- Oct, 2024 -
SiteCore Certified Developer
-Jan, 2003- Oct, 2024 -
Refactoring. A three day course by Martin Fowler.
-Jan, 2002- Oct, 2024
Experience
-
ViSenze
-
Singapore
-
Software Development
-
1 - 100 Employee
-
Principal Data Scientist, Discovery and Recommendations
-
Sep 2023 - Present
Helping with the technical planning and strategy building of the Recommender Systems, as well as, contributing. Provide leadership and mentoring in developing data science and analytics capabilities. Helping with the technical planning and strategy building of the Recommender Systems, as well as, contributing. Provide leadership and mentoring in developing data science and analytics capabilities.
-
-
-
Storytel
-
Sweden
-
Entertainment Providers
-
400 - 500 Employee
-
Recommender Systems Advisor
-
Jan 2023 - Present
Advising the StoryTel Data Science team on the evaluation, implementation and strategy of their recommender systems Advising the StoryTel Data Science team on the evaluation, implementation and strategy of their recommender systems
-
-
-
Shopify
-
Canada
-
Software Development
-
700 & Above Employee
-
Staff Recommender Engineer
-
Jan 2022 - Jun 2023
Subject matter expert in recommender systems at StoreFront Discovery Team at Shopify. I worked on different projects to add related, complementary and personalized recommendations across different surfaces. Key tasks: • Individual Contributor, building pipelines in Starscream and Python using PyG (Graph Neural Networks) and TensorFlow • Mentor and improve team towards state-of-the-art methods in Recommenders • Technical managing and mentoring of data scientists and engineers • Keeping up to date with current trends in RecSys • Defining the Recommender Systems north star, and strategy working towards it. Show less
-
-
-
-
Senior Data Scientist, Recommendations
-
Mar 2021 - Jan 2022
Worked in the RTL+ recommender team for recommending and personalising content in their new streaming platform, offering different types of content. Key tasks: • Implementation of personalization and recommender systems pipeline in Python on Google Cloud • Technical Lead and Strategy • Mentoring younger data scientists. Worked in the RTL+ recommender team for recommending and personalising content in their new streaming platform, offering different types of content. Key tasks: • Implementation of personalization and recommender systems pipeline in Python on Google Cloud • Technical Lead and Strategy • Mentoring younger data scientists.
-
-
-
IKEA
-
Netherlands
-
Retail
-
700 & Above Employee
-
Senior Data Scientist
-
Sep 2019 - Mar 2021
Implemented a Deep Reinforcement Learning agent to personalize promotions, a pipeline building Item2Vec embeddings and trained a sequence aware recommender system in GCP. Implemented a Deep Reinforcement Learning agent to personalize promotions, a pipeline building Item2Vec embeddings and trained a sequence aware recommender system in GCP.
-
-
-
LiveIntent, Inc.
-
United States
-
Advertising Services
-
100 - 200 Employee
-
Senior Data Scientist
-
Jun 2018 - Aug 2019
Working in a small team of data scientists on big data sets. Focused on Machine learning and Data analysis with Spark and Scalding running in AWS clusters. Using Python, Scala, Spark/Scalding. I was in charge of the retargeting part, focusing on predicting user intent and calculating recommendations Working in a small team of data scientists on big data sets. Focused on Machine learning and Data analysis with Spark and Scalding running in AWS clusters. Using Python, Scala, Spark/Scalding. I was in charge of the retargeting part, focusing on predicting user intent and calculating recommendations
-
-
-
Manning Publications Co.
-
United States
-
Book and Periodical Publishing
-
1 - 100 Employee
-
Author - Practical Recommender Systems
-
Jan 2015 - Dec 2018
Recommender systems are everywhere, helping you find everything from movies to jobs, restaurants to hospitals, even romance. Using behavioral and demographic data, these systems make predictions about what users will be most interested in at a particular time, resulting in high-quality, ordered, personalized suggestions. These systems are practically a necessity for keeping your site content current, useful, and interesting to your visitors. Practical Recommender Systems goes behind the curtain to show you how recommender systems work and, more importantly, how to create and apply them for your site. After you've covered the basics of how recommender systems work, you'll discover how to collect user data and produce personalized recommendations. Next, you'll learn how and where to use the most popular recommendation algorithms and see examples of them in action on sites like Amazon and Netflix. Finally, this hands-on guide covers scaling problems and other issues you may encounter as your site grows. www.manning.com/falk Show less
-
-
-
Karnov Group
-
Sweden
-
Information Technology & Services
-
1 - 100 Employee
-
Lead Data Scientist
-
Mar 2017 - Jun 2018
Worked on adding intelligence to the Karnov content platform which provides legal workers access to 150 years of Danish legal content. Our tasks were to optimize both the import pipeline but most importantly make the product more intelligent and personalized. We work with NLP, Python, Keras, TensorFlow, SpaCy, Sql. Key accomplishments: • Danish Language Model using the SpaCy framework • Named Entity Recognition (NER) model • Building a verdict classifier using Keras RNN neural network Show less
-
-
-
-
blogger
-
Jan 2014 - Jan 2018
Blogging in Danish mostly about Big data, Machine Learning and NoSql. Blogging in Danish mostly about Big data, Machine Learning and NoSql.
-
-
-
Adform
-
Design
-
1 - 100 Employee
-
Data scientist
-
Jan 2016 - Feb 2017
Part of the research team, focusing on recommender systems to show better e-shop ads, and improve forecasts and predictions to provide a programmatic advertising platform for publishers. Worked with Python, Sql, Vertica, Spark, Hadroop, Scala. Key accomplishments: • Content-based recommender prototype to add relevant ads. • Evaluation scheme for forecasting predictions Part of the research team, focusing on recommender systems to show better e-shop ads, and improve forecasts and predictions to provide a programmatic advertising platform for publishers. Worked with Python, Sql, Vertica, Spark, Hadroop, Scala. Key accomplishments: • Content-based recommender prototype to add relevant ads. • Evaluation scheme for forecasting predictions
-
-
-
Udacity
-
United States
-
E-learning
-
700 & Above Employee
-
Udacity Code Reviewer
-
Feb 2015 - Jan 2017
I review student project submissions. For each submission, I do both a thorough code review and project evaluation. I strive to give actionable and helpful feedback to students while also improving my code reviewing skills at the same time. I review student project submissions. For each submission, I do both a thorough code review and project evaluation. I strive to give actionable and helpful feedback to students while also improving my code reviewing skills at the same time.
-
-
-
-
Technical Product Manager, Machine Learning
-
Apr 2015 - Dec 2015
Implementing machine-learning features in Sitecore, part of the new analytics solution to scale for bigcustomers (Sitecore 7.5), content testing (Sitecore 8). In small SCRUM team. Using C# (4.5), MongoDb, Sql Server 2008, NUnit, TFS. Spark, Python.Key accomplishments:• Team Lead; track record of delivering scope on time.• Introduced Machine learning in content testing (MV and AB testing). Statistical relevancy Pearson Chi-squared K-means clustering.• Performance optimization of MongoDb and SQL server. Show less
-
-
Lead Developer
-
Sep 2012 - Apr 2015
Working with data collection and analytics.Key accomplishments• Team Lead; track record of delivering scope on time.• Took part in implementing a scalable analytics platform.using C#, MongoDb, Sql Server.
-
-
-
-
Recommender Engineer
-
Jan 2010 - Sep 2012
Working within a small team of developers implementing and extending a SaaS recommendation system, used primarily in the entertainment industry. Using C# (4.0), SQL server 2008, MongoDb, Asp.net MVC, Nunit, TFS and JavaScript, Elasticsearch. Occasional Scrum master. Building and maintaining a recommender system using Bayesian statistics, collaborative filtering and charting. Working within a small team of developers implementing and extending a SaaS recommendation system, used primarily in the entertainment industry. Using C# (4.0), SQL server 2008, MongoDb, Asp.net MVC, Nunit, TFS and JavaScript, Elasticsearch. Occasional Scrum master. Building and maintaining a recommender system using Bayesian statistics, collaborative filtering and charting.
-
-
-
Gesi srl
-
Italy
-
Oil and Gas
-
1 - 100 Employee
-
Lead/Senior Software Developer
-
Feb 2007 - Jan 2010
• C# (2.0) developer in Web Service (ASP.NET) project, implementing a central access gate for patient documents (EPR). A SOA project for the Basilicata region (Italy) based on ebXML and the DHE technology. • Implemented a VB.NET (2.0) proxy client to facilitate communication with the DHE (Distributed Healthcare Environment). • Implemented a database IIS server side caching component used by C#/ASP.NET (2.0) web clients. • Customer workshops to define the project. • C# (2.0) developer in Web Service (ASP.NET) project, implementing a central access gate for patient documents (EPR). A SOA project for the Basilicata region (Italy) based on ebXML and the DHE technology. • Implemented a VB.NET (2.0) proxy client to facilitate communication with the DHE (Distributed Healthcare Environment). • Implemented a database IIS server side caching component used by C#/ASP.NET (2.0) web clients. • Customer workshops to define the project.
-
-
-
TietoEnator A/S
-
Italy
-
Technology, Information and Internet
-
Senior Consultant
-
Mar 2004 - Jan 2007
• .NET server side design and development. • Leading the definition and introduction of the release management process. • Integration against DHE (Distributed Healthcare Environment). • Technical project management. • J2EE server side development on large SOA project for Copenhagen County (EPR). • Database modelling of Patient Data. • Responsible for Java education in our department. • Responsible for creating internal workshops and meetings on new technology. • Stationed 5 months at IBM in Aarhus Denmark representing the Copenhagen hospital society. • Integrating the Clinical process solution (Danish electronic patient journal) with DHE. • Mapped the GESI Distributed Healthcare Environment data model(based on the HISA) to The Danish general electronic patient journal (GEPJ) data model. Stationed 3 months at GESI in Rome, Italia Show less
-
-
-
Enzym A/S
-
Belgium
-
Mining
-
Software Developer
-
Nov 2003 - Mar 2004
* Building ASP.NET (1.0) based pages using the SiteCore CMS. * C#/ASP.Net development. * XSLT * Customer Workshops. * Building ASP.NET (1.0) based pages using the SiteCore CMS. * C#/ASP.Net development. * XSLT * Customer Workshops.
-
-
-
Mjølner Informatics
-
Denmark
-
Software Development
-
100 - 200 Employee
-
Student programmer
-
Aug 1998 - Feb 2002
Part of the BETA group, responsible for a part of the Mjolner IDE. Another task was to add .NET knowledge to my colleagues and I. Part of the BETA group, responsible for a part of the Mjolner IDE. Another task was to add .NET knowledge to my colleagues and I.
-
-
Education
-
Københavns Universitet
Statistical Method in Machine Learning -
Aarhus University
M.Sc., Master of Science, Computer Science; Information Technology -
Udacity
Nanodegree, Deep Reinforcement Learning -
Aarhus University
Supplementary degree, Mathematics -
Aarhus Katedralskole
Stud., Mat-fys
Community
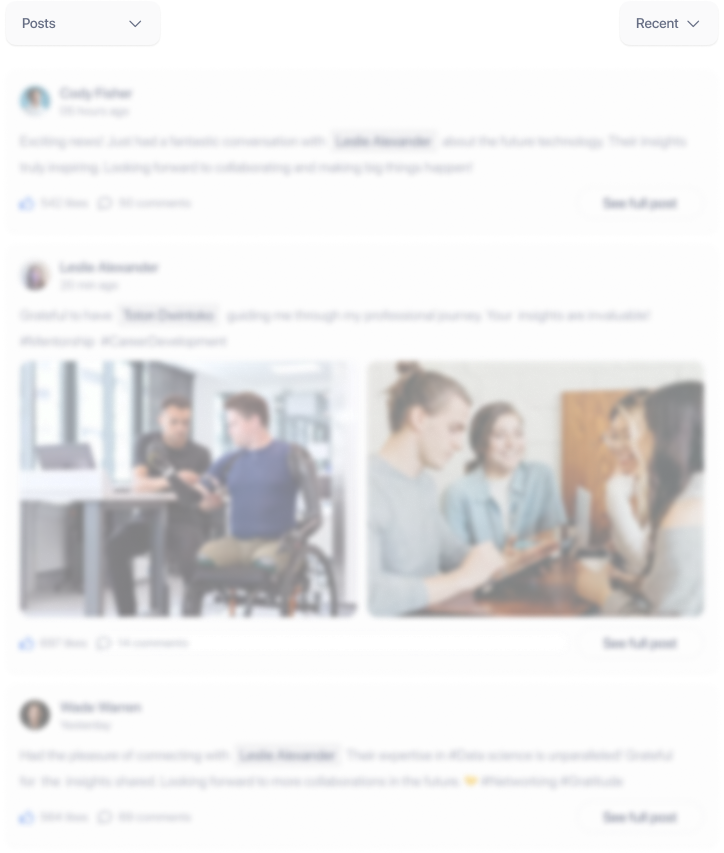