Jingxing (Joe) Qian
Team Advisor at aUToronto- Claim this Profile
Click to upgrade to our gold package
for the full feature experience.
-
English Full professional proficiency
-
Chinese (Simplified) Native or bilingual proficiency
Topline Score
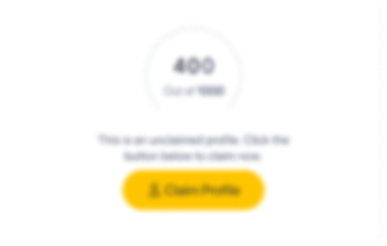
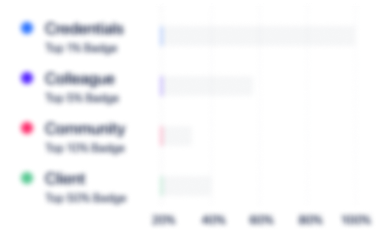
Bio


Experience
-
aUToronto
-
Canada
-
Automotive
-
1 - 100 Employee
-
Team Advisor
-
Jun 2021 - Present
aUToronto to compete in GM/SAE AutoDrive Challenge II
-
-
Team Lead
-
Jun 2020 - Jun 2021
aUToronto won 1st place in the final year of the GM/SAE AutoDrive Challenge!Oversaw high-level design decisions, managed both technical and non-technical squads, led system-level testing, and heavily contributed to the vehicle's perception systems.
-
-
On Leave
-
Aug 2019 - Jun 2020
-
-
Planning & Control Co-Lead
-
Aug 2018 - Aug 2019
aUToronto won 1st place in the second year of the GM/SAE AutoDrive Challenge!Co-led the Motion Planning & Control group in the University of Toronto's self-driving car team to design and develop a level 4 autonomous car and compete in the 4-year GM/SAE Autodrive challenge
-
-
Software Developer
-
Aug 2017 - Aug 2018
Developed trajectory tracking control algorithms for the vehicle
-
-
-
Zebra Technologies
-
United States
-
IT Services and IT Consulting
-
700 & Above Employee
-
Robotics Engineering Intern (Autonomous Navigation)
-
May 2017 - Aug 2018
R&D focused on mapping, localization and state estimation for the SmartSight mobile robot R&D focused on mapping, localization and state estimation for the SmartSight mobile robot
-
-
-
Learning Systems and Robotics Lab
-
Germany
-
Research
-
1 - 100 Employee
-
Summer Research Fellow
-
May 2016 - Sep 2016
Designing and tuning classical controllers to achieve precise trajectory tracking can be time-consuming and difficult for complex systems due to the high degrees of freedom and non-linearity of the dynamics. The group of four investigated and implemented methods that improve trajectory tracking control performance of unmanned aerial vehicle (quadcopters) through deep learning approaches. Given the desired trajectory, the deep neural network manipulates the input to the classical controller based on learned experience, compensating the controller's tracking error. The work was presented at the IEEE International Conference on Robotics and Automation (ICRA) 2017.
-
-
Education
-
University of Toronto
Doctor of Philosophy - PhD, Robotics -
University of Toronto
Master of Applied Science (MASc), Robotics -
University of Toronto
Bachelor of Applied Science (BASc), High Honours, Engineering Science Major in Robotics
Community
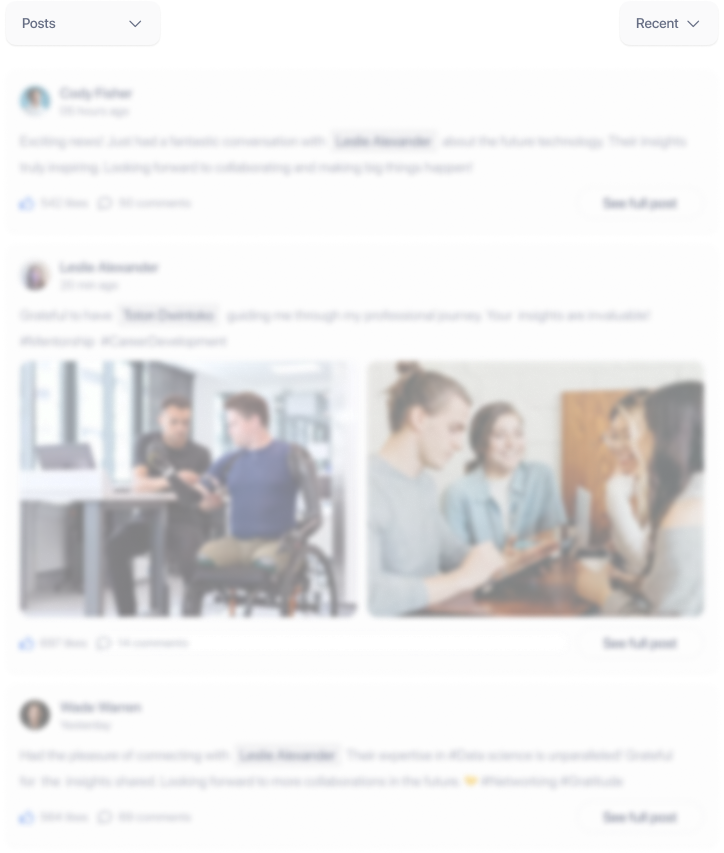