Jose Quesada
Founder, CEO at Data Science Retreat- Claim this Profile
Click to upgrade to our gold package
for the full feature experience.
-
English Native or bilingual proficiency
-
Spanish Native or bilingual proficiency
-
German Elementary proficiency
-
Portuguese Limited working proficiency
Topline Score
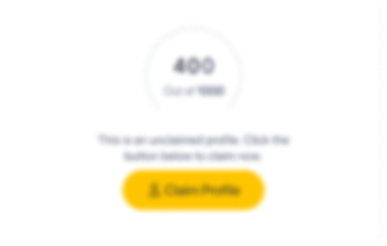
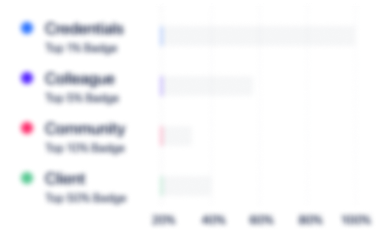
Bio

Peter Guggi
Among other things, I help clients writing a solid presentation for investors. When we arrive to customer lifetime value (CLV), we have to go with best guesses. This is a pity because this is THE most telling number to estimate the value of a company, not to mention an incredible tool for daily operations. It's certainly a number that sophisticated investors want to know, and one that founders should have at the top of their minds. When I realized that CLV nowadays can be calculated with precision (10% error), I was blown away. Jose uses all kinds of techniques, from advanced probabilistic models to growth hacking tricks, to make things happen. Based on the way he first hoovers over problems to then dissect and methodically analyze them, issue by issue, I can see how his services are in demand. From my experience, he can bring significant value to any business, maybe more so the larger the business is and the more detailed their history of purchases is

Frank van Harmelen
Jose and I collaborated in the EU funded research project LarKC, building the Large Knowledge Collider, an infrastructure for very large scale reasoning with data on the Web. One of the exciting aspects of that project was the collaboration between computer scientists and cognitive scientists. Jose was absolutely crucial in bridging the gap between those two communities. I remember very well the first meeting where he attended. I assumed he was a psychologist (since he came from MPI), but he kept asking all these hard-core techie questions! Since that first meeting, Jose has continued to impress me with his breadth of knowledge and skills that span different disciplines. He has also impressed me with his creativity in experimental design, with his perseverance in the face of experimental difficulties, and with his intellectual honesty and integrity. It earned him a comment from the project reviewers that they found it refreshing to see somebody give a lucid analysis of negative experimental results, and that they found that analysis more insightful than yet another haleluja-report. I can warmly recommend Jose as a creative and highly capable scientist.

Peter Guggi
Among other things, I help clients writing a solid presentation for investors. When we arrive to customer lifetime value (CLV), we have to go with best guesses. This is a pity because this is THE most telling number to estimate the value of a company, not to mention an incredible tool for daily operations. It's certainly a number that sophisticated investors want to know, and one that founders should have at the top of their minds. When I realized that CLV nowadays can be calculated with precision (10% error), I was blown away. Jose uses all kinds of techniques, from advanced probabilistic models to growth hacking tricks, to make things happen. Based on the way he first hoovers over problems to then dissect and methodically analyze them, issue by issue, I can see how his services are in demand. From my experience, he can bring significant value to any business, maybe more so the larger the business is and the more detailed their history of purchases is

Frank van Harmelen
Jose and I collaborated in the EU funded research project LarKC, building the Large Knowledge Collider, an infrastructure for very large scale reasoning with data on the Web. One of the exciting aspects of that project was the collaboration between computer scientists and cognitive scientists. Jose was absolutely crucial in bridging the gap between those two communities. I remember very well the first meeting where he attended. I assumed he was a psychologist (since he came from MPI), but he kept asking all these hard-core techie questions! Since that first meeting, Jose has continued to impress me with his breadth of knowledge and skills that span different disciplines. He has also impressed me with his creativity in experimental design, with his perseverance in the face of experimental difficulties, and with his intellectual honesty and integrity. It earned him a comment from the project reviewers that they found it refreshing to see somebody give a lucid analysis of negative experimental results, and that they found that analysis more insightful than yet another haleluja-report. I can warmly recommend Jose as a creative and highly capable scientist.

Peter Guggi
Among other things, I help clients writing a solid presentation for investors. When we arrive to customer lifetime value (CLV), we have to go with best guesses. This is a pity because this is THE most telling number to estimate the value of a company, not to mention an incredible tool for daily operations. It's certainly a number that sophisticated investors want to know, and one that founders should have at the top of their minds. When I realized that CLV nowadays can be calculated with precision (10% error), I was blown away. Jose uses all kinds of techniques, from advanced probabilistic models to growth hacking tricks, to make things happen. Based on the way he first hoovers over problems to then dissect and methodically analyze them, issue by issue, I can see how his services are in demand. From my experience, he can bring significant value to any business, maybe more so the larger the business is and the more detailed their history of purchases is

Frank van Harmelen
Jose and I collaborated in the EU funded research project LarKC, building the Large Knowledge Collider, an infrastructure for very large scale reasoning with data on the Web. One of the exciting aspects of that project was the collaboration between computer scientists and cognitive scientists. Jose was absolutely crucial in bridging the gap between those two communities. I remember very well the first meeting where he attended. I assumed he was a psychologist (since he came from MPI), but he kept asking all these hard-core techie questions! Since that first meeting, Jose has continued to impress me with his breadth of knowledge and skills that span different disciplines. He has also impressed me with his creativity in experimental design, with his perseverance in the face of experimental difficulties, and with his intellectual honesty and integrity. It earned him a comment from the project reviewers that they found it refreshing to see somebody give a lucid analysis of negative experimental results, and that they found that analysis more insightful than yet another haleluja-report. I can warmly recommend Jose as a creative and highly capable scientist.

Peter Guggi
Among other things, I help clients writing a solid presentation for investors. When we arrive to customer lifetime value (CLV), we have to go with best guesses. This is a pity because this is THE most telling number to estimate the value of a company, not to mention an incredible tool for daily operations. It's certainly a number that sophisticated investors want to know, and one that founders should have at the top of their minds. When I realized that CLV nowadays can be calculated with precision (10% error), I was blown away. Jose uses all kinds of techniques, from advanced probabilistic models to growth hacking tricks, to make things happen. Based on the way he first hoovers over problems to then dissect and methodically analyze them, issue by issue, I can see how his services are in demand. From my experience, he can bring significant value to any business, maybe more so the larger the business is and the more detailed their history of purchases is

Frank van Harmelen
Jose and I collaborated in the EU funded research project LarKC, building the Large Knowledge Collider, an infrastructure for very large scale reasoning with data on the Web. One of the exciting aspects of that project was the collaboration between computer scientists and cognitive scientists. Jose was absolutely crucial in bridging the gap between those two communities. I remember very well the first meeting where he attended. I assumed he was a psychologist (since he came from MPI), but he kept asking all these hard-core techie questions! Since that first meeting, Jose has continued to impress me with his breadth of knowledge and skills that span different disciplines. He has also impressed me with his creativity in experimental design, with his perseverance in the face of experimental difficulties, and with his intellectual honesty and integrity. It earned him a comment from the project reviewers that they found it refreshing to see somebody give a lucid analysis of negative experimental results, and that they found that analysis more insightful than yet another haleluja-report. I can warmly recommend Jose as a creative and highly capable scientist.

Experience
-
Data Science Retreat
-
Germany
-
Professional Training and Coaching
-
1 - 100 Employee
-
Founder, CEO
-
Nov 2013 - Present
Data Science Retreat (DSR) is the longest-running data science training provider in Europe. 1. It offers an advanced level curriculum taught by experts from the industry who have excelled in Data Science and Machine Learning field and not in-house teachers. This ensures the curriculum is fresh and much deeper than when taught by teachers with no real world experience. 2. Our students will be trained in topics that will be in the market in two years; placing them ahead of the curve. Not… Show more Data Science Retreat (DSR) is the longest-running data science training provider in Europe. 1. It offers an advanced level curriculum taught by experts from the industry who have excelled in Data Science and Machine Learning field and not in-house teachers. This ensures the curriculum is fresh and much deeper than when taught by teachers with no real world experience. 2. Our students will be trained in topics that will be in the market in two years; placing them ahead of the curve. Not topics that target the immediate needs of companies now (which will be obsolete soon because the field moves very fast!). Advanced topics such as Deep Learning, Transfer Learning, NLP, Computer vision with pytorch and tensorflow, Reinforcement Learning, Models in production and practical aspects of data science are taught in depth which will better highlight the participants profile in the job market. 3. By the end of the program participants will be skilled enough to develop an original data science product prototype all by their own which is the signature of our program. These projects make them unique in the market. Our graduates get associate level positions which pay a higher salary than an entry level or junior data scientist level position. Some of our trainees reached C level and VP level positions. Therefore the return on investment is higher compared to more basic trainings. Show less Data Science Retreat (DSR) is the longest-running data science training provider in Europe. 1. It offers an advanced level curriculum taught by experts from the industry who have excelled in Data Science and Machine Learning field and not in-house teachers. This ensures the curriculum is fresh and much deeper than when taught by teachers with no real world experience. 2. Our students will be trained in topics that will be in the market in two years; placing them ahead of the curve. Not… Show more Data Science Retreat (DSR) is the longest-running data science training provider in Europe. 1. It offers an advanced level curriculum taught by experts from the industry who have excelled in Data Science and Machine Learning field and not in-house teachers. This ensures the curriculum is fresh and much deeper than when taught by teachers with no real world experience. 2. Our students will be trained in topics that will be in the market in two years; placing them ahead of the curve. Not topics that target the immediate needs of companies now (which will be obsolete soon because the field moves very fast!). Advanced topics such as Deep Learning, Transfer Learning, NLP, Computer vision with pytorch and tensorflow, Reinforcement Learning, Models in production and practical aspects of data science are taught in depth which will better highlight the participants profile in the job market. 3. By the end of the program participants will be skilled enough to develop an original data science product prototype all by their own which is the signature of our program. These projects make them unique in the market. Our graduates get associate level positions which pay a higher salary than an entry level or junior data scientist level position. Some of our trainees reached C level and VP level positions. Therefore the return on investment is higher compared to more basic trainings. Show less
-
-
-
AI Deep DIve
-
Toronto, Canada Area
-
Founder and CEO
-
Jan 2019 - Jan 2020
AI Deep Dive was training that delivers proof-of-concept AI products. We ran a full-time immersive program for career-switchers (3 months) and 1-week courses for companies. On our immersive course, we offered more than 250 hours of instruction from recognized leaders in the field. Teachers cover only the topics they are masters of. Most mentors/teachers are leading industry practitioners, and they teach only the topic they have mastered. We trained you to produce… Show more AI Deep Dive was training that delivers proof-of-concept AI products. We ran a full-time immersive program for career-switchers (3 months) and 1-week courses for companies. On our immersive course, we offered more than 250 hours of instruction from recognized leaders in the field. Teachers cover only the topics they are masters of. Most mentors/teachers are leading industry practitioners, and they teach only the topic they have mastered. We trained you to produce solid models that work in production; with monitoring and reliable metrics front and center. You create an original portfolio project that you present to companies on a demo day. In the past, several of these projects had a potential for social impact. On our company training, our instructors and consultants emphasized practical, sustainable skills that ease your AI transformation. AI Deep Dive can also provide AI strategy and implementation for companies that don't necessarily have lots of data. We provided training for your engineers and managers so that, with our help, let you build a feature with AI capability that you thought was not possible before. Off-the-shelf tools are fantastically powerful (for example, pretrained models); we taught you to wield them and develop products or features for your product. Show less AI Deep Dive was training that delivers proof-of-concept AI products. We ran a full-time immersive program for career-switchers (3 months) and 1-week courses for companies. On our immersive course, we offered more than 250 hours of instruction from recognized leaders in the field. Teachers cover only the topics they are masters of. Most mentors/teachers are leading industry practitioners, and they teach only the topic they have mastered. We trained you to produce… Show more AI Deep Dive was training that delivers proof-of-concept AI products. We ran a full-time immersive program for career-switchers (3 months) and 1-week courses for companies. On our immersive course, we offered more than 250 hours of instruction from recognized leaders in the field. Teachers cover only the topics they are masters of. Most mentors/teachers are leading industry practitioners, and they teach only the topic they have mastered. We trained you to produce solid models that work in production; with monitoring and reliable metrics front and center. You create an original portfolio project that you present to companies on a demo day. In the past, several of these projects had a potential for social impact. On our company training, our instructors and consultants emphasized practical, sustainable skills that ease your AI transformation. AI Deep Dive can also provide AI strategy and implementation for companies that don't necessarily have lots of data. We provided training for your engineers and managers so that, with our help, let you build a feature with AI capability that you thought was not possible before. Off-the-shelf tools are fantastically powerful (for example, pretrained models); we taught you to wield them and develop products or features for your product. Show less
-
-
-
Deep Learning Retreat
-
United States
-
Professional Training and Coaching
-
Founder
-
Jul 2017 - Dec 2017
Deep learning Retreat (DLR) was the first deep learning school in the world. We trained people who want to either: - Have a job in a tech company - Have tons of impact by going alone on their own startup or doing ‘good’ We made sure we fulfilled those two customer needs by giving them the best environment to produce an impressive portfolio project. Deep learning Retreat (DLR) was the first deep learning school in the world. We trained people who want to either: - Have a job in a tech company - Have tons of impact by going alone on their own startup or doing ‘good’ We made sure we fulfilled those two customer needs by giving them the best environment to produce an impressive portfolio project.
-
-
-
Hacker Retreat
-
Berlin Area, Germany
-
Founder and Facilitator
-
Oct 2013 - Jul 2014
Hacker retreat is an 'exclusive club' whose mission is to make you a better programmer. It happens in Berlin, brings mentors and students from all over the world, and lasts about three months. The ideal Hacker Retreater is bright, curious, and kind. She doesn't necessarily have a significant amount of work experience (though many Retreaters are fluent in several programming languages and have done serious projects!), but she knows enough to know 1) what she doesn't know, 2) how to learn… Show more Hacker retreat is an 'exclusive club' whose mission is to make you a better programmer. It happens in Berlin, brings mentors and students from all over the world, and lasts about three months. The ideal Hacker Retreater is bright, curious, and kind. She doesn't necessarily have a significant amount of work experience (though many Retreaters are fluent in several programming languages and have done serious projects!), but she knows enough to know 1) what she doesn't know, 2) how to learn more, and 3) that she loves to program in a fundamental way. We help companies filling hard-to-find positions, because by definition 'those who are never looking for a job' feel attracted to an environment where everyone is hell-bent trying to improve themselves. Show less Hacker retreat is an 'exclusive club' whose mission is to make you a better programmer. It happens in Berlin, brings mentors and students from all over the world, and lasts about three months. The ideal Hacker Retreater is bright, curious, and kind. She doesn't necessarily have a significant amount of work experience (though many Retreaters are fluent in several programming languages and have done serious projects!), but she knows enough to know 1) what she doesn't know, 2) how to learn… Show more Hacker retreat is an 'exclusive club' whose mission is to make you a better programmer. It happens in Berlin, brings mentors and students from all over the world, and lasts about three months. The ideal Hacker Retreater is bright, curious, and kind. She doesn't necessarily have a significant amount of work experience (though many Retreaters are fluent in several programming languages and have done serious projects!), but she knows enough to know 1) what she doesn't know, 2) how to learn more, and 3) that she loves to program in a fundamental way. We help companies filling hard-to-find positions, because by definition 'those who are never looking for a job' feel attracted to an environment where everyone is hell-bent trying to improve themselves. Show less
-
-
-
Jose Quesada
-
Berlin Area, Germany
-
Principal Data scientist
-
Jan 2013 - Apr 2014
Contact me if you want to know your Customer Lifetime Value, and a single percent point error could be very costly. Recent advances in predictive models are truly revolutionary. Allocating acquisition and retention budgets 'by ear' will be as archaic as horse carriages soon. This change is not widespread yet, so there's a big opportunity for businesses to leapfrog the competition. Contact me if you want to know your Customer Lifetime Value, and a single percent point error could be very costly. Recent advances in predictive models are truly revolutionary. Allocating acquisition and retention budgets 'by ear' will be as archaic as horse carriages soon. This change is not widespread yet, so there's a big opportunity for businesses to leapfrog the competition.
-
-
-
GetYourGuide
-
Germany
-
Software Development
-
500 - 600 Employee
-
Data Scientist
-
Aug 2013 - Nov 2013
Senior data scientist. Responsibilities include: - Deep segmentation - Valuing adwords campaigns (building on top of my existing CLV models) - Integrating information on intent, onsite behavior, purchase, and inventory changes - Retargeting - Email marketing Senior data scientist. Responsibilities include: - Deep segmentation - Valuing adwords campaigns (building on top of my existing CLV models) - Integrating information on intent, onsite behavior, purchase, and inventory changes - Retargeting - Email marketing
-
-
-
Memoryous
-
Berlin Area, Germany
-
Founder
-
Jul 2011 - May 2013
Founder and CEO. Memoryous is a tool for accelerated-learning of programming languages. Memoryous computes the optimal repetition pattern and presents question/answer pairs. I wrote the business plan, got eXist funding, and managed a 8-people team. Memoryous uses technology from memory research (retention curves, with optimal parameters per user) and machine learning (LSA). Founder and CEO. Memoryous is a tool for accelerated-learning of programming languages. Memoryous computes the optimal repetition pattern and presents question/answer pairs. I wrote the business plan, got eXist funding, and managed a 8-people team. Memoryous uses technology from memory research (retention curves, with optimal parameters per user) and machine learning (LSA).
-
-
-
Fluid shopping
-
Berlin
-
Chief Happiness Officer
-
Mar 2012 - Dec 2012
Most companies have a 'gut feeling' of what their customer lifetime value (CLV) is, but no way of knowing for sure. Yet this is the number that would make the largest impact to their bottom line, if only it was possible to have it. Turns out CLV can be computed with surprising accuracy (around 10% error) at the individual level. Probabilistic marketing models in a non-contractual setting, such as retail or gaming, assume each customer has two variables - one for how often he orders, and another… Show more Most companies have a 'gut feeling' of what their customer lifetime value (CLV) is, but no way of knowing for sure. Yet this is the number that would make the largest impact to their bottom line, if only it was possible to have it. Turns out CLV can be computed with surprising accuracy (around 10% error) at the individual level. Probabilistic marketing models in a non-contractual setting, such as retail or gaming, assume each customer has two variables - one for how often he orders, and another for how long he’ll last. These models are far more accurate than say cohort analyses approaches. Since you are going to bet money on these predictions, minimizing error is crucial. From my experience, the probabilistic models I use are around 5%-12% error. They enable you to zoom in on a specific user that is one or two months old and to make a projected CLV calculation for that individual user. You can compute CLV for a product category, traffic source or any other segment. Show less Most companies have a 'gut feeling' of what their customer lifetime value (CLV) is, but no way of knowing for sure. Yet this is the number that would make the largest impact to their bottom line, if only it was possible to have it. Turns out CLV can be computed with surprising accuracy (around 10% error) at the individual level. Probabilistic marketing models in a non-contractual setting, such as retail or gaming, assume each customer has two variables - one for how often he orders, and another… Show more Most companies have a 'gut feeling' of what their customer lifetime value (CLV) is, but no way of knowing for sure. Yet this is the number that would make the largest impact to their bottom line, if only it was possible to have it. Turns out CLV can be computed with surprising accuracy (around 10% error) at the individual level. Probabilistic marketing models in a non-contractual setting, such as retail or gaming, assume each customer has two variables - one for how often he orders, and another for how long he’ll last. These models are far more accurate than say cohort analyses approaches. Since you are going to bet money on these predictions, minimizing error is crucial. From my experience, the probabilistic models I use are around 5%-12% error. They enable you to zoom in on a specific user that is one or two months old and to make a projected CLV calculation for that individual user. You can compute CLV for a product category, traffic source or any other segment. Show less
-
-
-
Max Planck Institute for Human Development
-
Germany
-
Research Services
-
100 - 200 Employee
-
Researcher
-
Jun 2008 - Nov 2011
I work on algorithms and implementation of semantic web technology. To know more about the project, see: www.larkc.eu Larkc is platform for massive distributed incomplete reasoning that removes the scalability barriers of currently existing reasoning systems for the Semantic Web. That is, at the time it was the only system to do reasoning on the entire semantic web (Trillions of entries). I work on algorithms and implementation of semantic web technology. To know more about the project, see: www.larkc.eu Larkc is platform for massive distributed incomplete reasoning that removes the scalability barriers of currently existing reasoning systems for the Semantic Web. That is, at the time it was the only system to do reasoning on the entire semantic web (Trillions of entries).
-
-
-
Larkc
-
Berlin Area, Germany
-
Researcher
-
2008 - 2011
A problem with the semantic web is that you cannot reason with the entirety of it at once. And by reasoning, I mean 'running a query'. Imagine that your data are spread around different computers on different domains. They are all following a standard, and the query language you use will work, ... if only they were all reachable. Maybe one organization has the best database on drug interactions; another one has the best list of side effects, and yet another one has the best data on sympthoms… Show more A problem with the semantic web is that you cannot reason with the entirety of it at once. And by reasoning, I mean 'running a query'. Imagine that your data are spread around different computers on different domains. They are all following a standard, and the query language you use will work, ... if only they were all reachable. Maybe one organization has the best database on drug interactions; another one has the best list of side effects, and yet another one has the best data on sympthoms. They all use RDF to reprensent this knowledge. But running a query accross domains simply doesn't work. On top of that, given the sheer size of the semantic web (According to wikipedia, in September 2011 it was 31 billion RDF triples, interlinked by around 504 million RDF links) it will be difficult for a query engine to produce a result. In 2013, these numbers much be much higher if we count Google’s Knowledge Graph. Making algorithms that work on the entire Semantic Web the challenge we took with LarKC (2008-2011). The overall aim of LarKC was to build an integrated platform for semantic computing on a scale well beyond what was currently possible. The platform aims to fulfill needs in sectors that are dependent on massive heterogeneous information sources such as telecommunication services, bio-medical research, and drug-discovery. LarKC is based on a pluggable architecture in which it is possible to exploit techniques and heuristics from diverse areas such as databases, machine learning, cognitive science, Semantic Web, and others. As result LarKC allows to integrate logical reasoning with search methods. Show less A problem with the semantic web is that you cannot reason with the entirety of it at once. And by reasoning, I mean 'running a query'. Imagine that your data are spread around different computers on different domains. They are all following a standard, and the query language you use will work, ... if only they were all reachable. Maybe one organization has the best database on drug interactions; another one has the best list of side effects, and yet another one has the best data on sympthoms… Show more A problem with the semantic web is that you cannot reason with the entirety of it at once. And by reasoning, I mean 'running a query'. Imagine that your data are spread around different computers on different domains. They are all following a standard, and the query language you use will work, ... if only they were all reachable. Maybe one organization has the best database on drug interactions; another one has the best list of side effects, and yet another one has the best data on sympthoms. They all use RDF to reprensent this knowledge. But running a query accross domains simply doesn't work. On top of that, given the sheer size of the semantic web (According to wikipedia, in September 2011 it was 31 billion RDF triples, interlinked by around 504 million RDF links) it will be difficult for a query engine to produce a result. In 2013, these numbers much be much higher if we count Google’s Knowledge Graph. Making algorithms that work on the entire Semantic Web the challenge we took with LarKC (2008-2011). The overall aim of LarKC was to build an integrated platform for semantic computing on a scale well beyond what was currently possible. The platform aims to fulfill needs in sectors that are dependent on massive heterogeneous information sources such as telecommunication services, bio-medical research, and drug-discovery. LarKC is based on a pluggable architecture in which it is possible to exploit techniques and heuristics from diverse areas such as databases, machine learning, cognitive science, Semantic Web, and others. As result LarKC allows to integrate logical reasoning with search methods. Show less
-
-
-
University of Sussex
-
United Kingdom
-
Higher Education
-
700 & Above Employee
-
researcher
-
Jan 2006 - Jan 2007
-
-
-
Carnegie Mellon University
-
United States
-
Higher Education
-
700 & Above Employee
-
Research associate
-
2004 - 2005
-
-
-
-
researcher
-
2004 - 2005
-
-
-
University of Colorado Boulder
-
United States
-
Higher Education
-
700 & Above Employee
-
Researcher
-
2000 - 2004
I worked on my dissertation while keeping the public demo of our lab's premieer product (Latent Semantic Analysis LSA). I expanded the LSA framework to work on problem spaces. That means to go bottom-up from raw data on different problem solutions to a representation of the problem that might be what a human forms after lots of experience in a task. Take for example the task of landing a plane. Expert pilots know how to do it well; and a landing is a vector of states of the system… Show more I worked on my dissertation while keeping the public demo of our lab's premieer product (Latent Semantic Analysis LSA). I expanded the LSA framework to work on problem spaces. That means to go bottom-up from raw data on different problem solutions to a representation of the problem that might be what a human forms after lots of experience in a task. Take for example the task of landing a plane. Expert pilots know how to do it well; and a landing is a vector of states of the system (plane) changing over time. After many landings they have developed 'an intuition' of what is to make a good landing. I quantified this intuition as a vector space. During my time at UC Boulder I won highly-competitive grant budget to run experiments in a hi-fi flying simulator. This was my 'sci-fi' project, something that even my mentors at the time discarded as 'too good to be true'. The experiments did work though to everyone's surprise. I produced a machine that could rate landing quality as good as two human raters (expert pilots) could agree with each other. The system had immediate applied value, and UC Boulder offered me to pay for a patent for it. At the time I was not very commercially-minded (big believer in open source, open data etc) and I refused. In retrospect, I should have patented it. Show less I worked on my dissertation while keeping the public demo of our lab's premieer product (Latent Semantic Analysis LSA). I expanded the LSA framework to work on problem spaces. That means to go bottom-up from raw data on different problem solutions to a representation of the problem that might be what a human forms after lots of experience in a task. Take for example the task of landing a plane. Expert pilots know how to do it well; and a landing is a vector of states of the system… Show more I worked on my dissertation while keeping the public demo of our lab's premieer product (Latent Semantic Analysis LSA). I expanded the LSA framework to work on problem spaces. That means to go bottom-up from raw data on different problem solutions to a representation of the problem that might be what a human forms after lots of experience in a task. Take for example the task of landing a plane. Expert pilots know how to do it well; and a landing is a vector of states of the system (plane) changing over time. After many landings they have developed 'an intuition' of what is to make a good landing. I quantified this intuition as a vector space. During my time at UC Boulder I won highly-competitive grant budget to run experiments in a hi-fi flying simulator. This was my 'sci-fi' project, something that even my mentors at the time discarded as 'too good to be true'. The experiments did work though to everyone's surprise. I produced a machine that could rate landing quality as good as two human raters (expert pilots) could agree with each other. The system had immediate applied value, and UC Boulder offered me to pay for a patent for it. At the time I was not very commercially-minded (big believer in open source, open data etc) and I refused. In retrospect, I should have patented it. Show less
-
-
Education
-
University of Colorado Boulder
psychology, cognitive science -
Carnegie Mellon University
Cognitive science, post doc -
University of Warwick
Post doc with Nick Chater, cognitive science, decision making -
Max Planck Institute for Human Development
Postdoc, Web-scale reasoning and decision making -
Universidad de Granada
Bachelor of Science (BSc), Experimental Psychology
Community
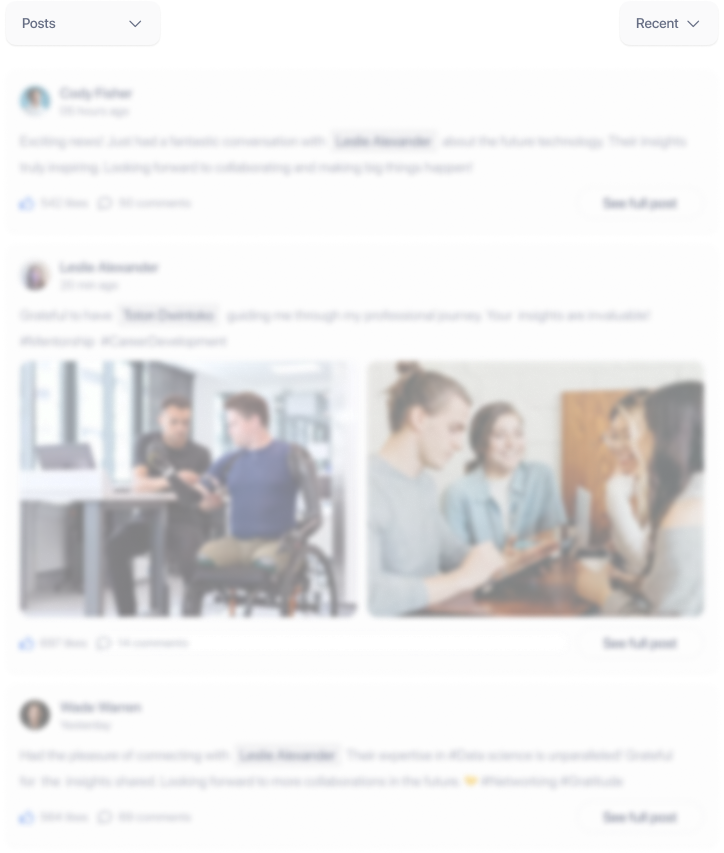