Praveen Radhakrishnan
Graduate Research Assistant at College of Natural Sciences, The University of Texas at Austin- Claim this Profile
Click to upgrade to our gold package
for the full feature experience.
Topline Score
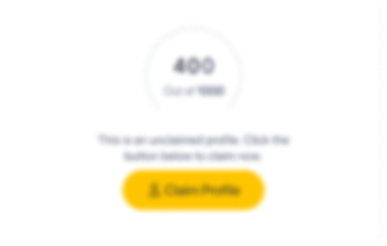
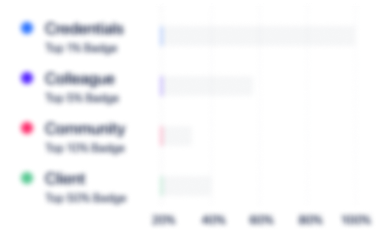
Bio


Experience
-
College of Natural Sciences, The University of Texas at Austin
-
United States
-
Higher Education
-
100 - 200 Employee
-
Graduate Research Assistant
-
Sep 2022 - Present
Generated insights that help CDC reduce flu transmission by developing Bayesian Hierarchical GLM models to estimate the impact of COVID-19 NPIs(Non-Pharm.Interventions) on Flu cases for U.S 50 states ◦ Improved the reliability of estimation of COVID-19 NPI impacts by identifying optimal lags and parameters using feature engineering, data processing, and statistical tests. Generated insights that help CDC reduce flu transmission by developing Bayesian Hierarchical GLM models to estimate the impact of COVID-19 NPIs(Non-Pharm.Interventions) on Flu cases for U.S 50 states ◦ Improved the reliability of estimation of COVID-19 NPI impacts by identifying optimal lags and parameters using feature engineering, data processing, and statistical tests.
-
-
-
Intelligent Environments Laboratory
-
Research Services
-
Research Assistant
-
Jan 2022 - Present
◦ Discovered findings which support Austin Energy to reduce outages in future extreme weather events by optimizing the recall score of anomaly detection pipeline by 35% using ensemble majority voting with Local Outlier Factor, K-means clustering, and LSTM-based Autoencoder with Self-Attention. ◦ Enabled better decision-making for future electricity market planning by enhancing the accuracy of the Random Forest pipeline by 18% through feature importance and nested cross-validation ◦ Discovered findings which support Austin Energy to reduce outages in future extreme weather events by optimizing the recall score of anomaly detection pipeline by 35% using ensemble majority voting with Local Outlier Factor, K-means clustering, and LSTM-based Autoencoder with Self-Attention. ◦ Enabled better decision-making for future electricity market planning by enhancing the accuracy of the Random Forest pipeline by 18% through feature importance and nested cross-validation
-
-
-
The University of Texas at Austin
-
United States
-
Higher Education
-
700 & Above Employee
-
Teaching Assistant
-
Jan 2022 - Jan 2023
1. CE 311K 2. CE 351 1. CE 311K 2. CE 351
-
-
Education
-
The University of Texas at Austin
Masters of Science in Engineering -
Vellore Institute of Technology
Bachelor of Technology, Civil Engineering
Community
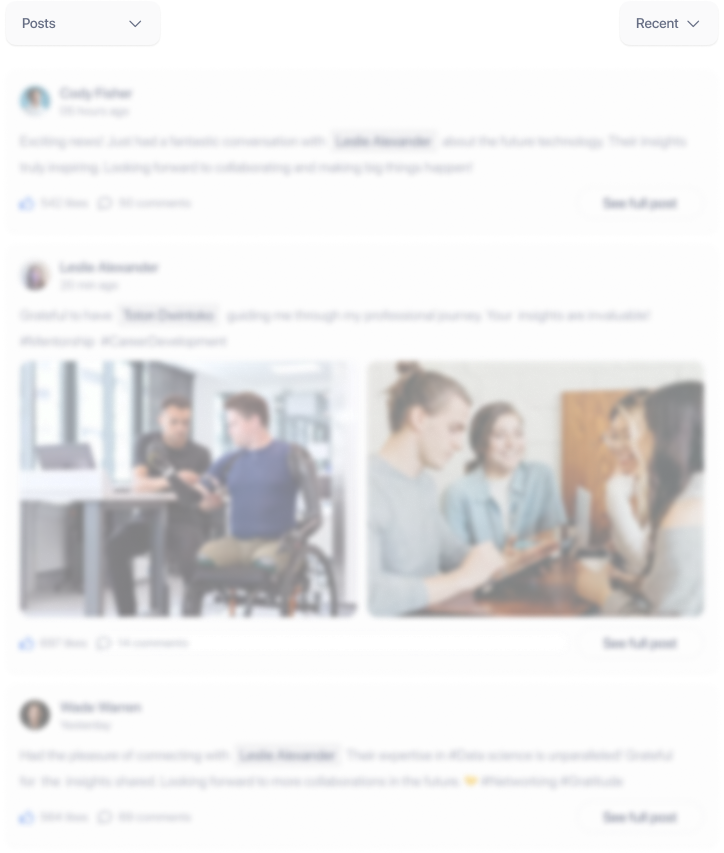