Ngoc Quang Luong
Senior Researcher at IDLab (UGent - UAntwerpen - imec)- Claim this Profile
Click to upgrade to our gold package
for the full feature experience.
-
English Full professional proficiency
-
French Full professional proficiency
-
Vietnamese Native or bilingual proficiency
-
Dutch Limited working proficiency
Topline Score
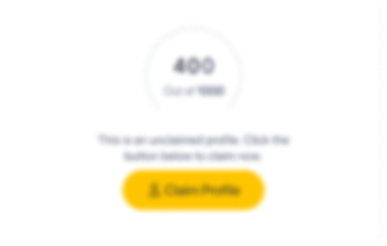
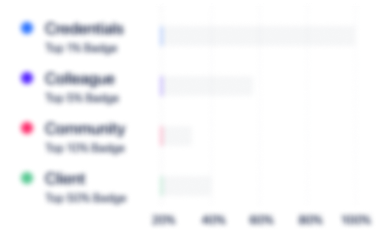
Bio


Credentials
-
Convolutional Neural Networks
CourseraSep, 2021- Nov, 2024 -
Deep Learning Specialization
CourseraSep, 2021- Nov, 2024 -
Structuring Machine Learning Projects
CourseraAug, 2021- Nov, 2024 -
Improving Deep Neural Networks: Hyperparameter Tuning, Regularization and Optimization
CourseraJul, 2021- Nov, 2024 -
Neural Networks and Deep Learning
CourseraJul, 2021- Nov, 2024 -
Sequence Models
CourseraAug, 2011- Nov, 2024 -
Certificate of the University Teacher’s Training Course on Web Development Technology
National Institute of Technical Teachers’ Training and Research, Bhopal, IndiaJan, 2011- Nov, 2024 -
Fundamental Engineer Certificate
VITEC (equivalent to Japan Fundamental Information Technology Skill Standards)Nov, 2006- Nov, 2024
Experience
-
IDLab (UGent - UAntwerpen - imec)
-
Belgium
-
Research
-
1 - 100 Employee
-
Senior Researcher
-
Apr 2022 - Present
I am participating in some European projects which offer AI-driven solutions in logistics, including COOCK SMART PORT (https://www.thebeacon.eu/innovation/projects/coock-smart-port-2025/) and PIONEERS (https://pioneers-ports.eu/). These projects tackle various operational bottlenecks of the current logistic chain, ranging from the deep-sea, short sea, intra-port services, to hinterland transportation networks. We are integrating data coming from multiple stakeholders and training predictive models to mitigate the scheduling margins, dwelling, delay and the suboptimal use of assets, which bring value to all stakeholders involved in the chain. Show less
-
-
-
Cerence Inc.
-
United States
-
Software Development
-
700 & Above Employee
-
Senior Research Engineer
-
Sep 2019 - Apr 2022
Research Deep Learning solutions for leveraging rich context in Question Answering and Dialog models. Extract and compress context from dialog history (user questions and/or interpretations) Combine the history with the current context of the selected template in order to generated grammatically-correct and natural textual output at runtime. Continuous learning: Employ the predicted values to train the embedded model (inside the car, when connectivity is not guaranteed or low latency is needed) Tune pretrained DL models (e.g. BERT) using in-domain logged data to tackle language specific issues (mainly applied for English and German) Show less
-
-
-
Nuance Communications
-
United States
-
Software Development
-
700 & Above Employee
-
Senior NLP Research Engineer
-
Feb 2017 - Apr 2022
• Conduct Machine Learning and Data Clustering methods to prepare training data for Text Normalization. • Implement grammatical and morphological agreement in Natural Language Generation outputs. • Conduct Machine Learning and Data Clustering methods to prepare training data for Text Normalization. • Implement grammatical and morphological agreement in Natural Language Generation outputs.
-
-
-
Idiap Research Institute
-
Switzerland
-
Research
-
100 - 200 Employee
-
Postdoctoral Researcher
-
Dec 2014 - Dec 2016
I joined MODERN project since December 2014. My main task, as defined in the project report, is to exploit Discourse Entity and Relation model to enhance Statistical Machine Translation (SMT) decoder. So far, my work lies principally on the use of discourse information and morphological features for enhancing the quality of pronoun translation, specifically applying for English – French language pair. The research can be divided into three sub-missions, mainly based on the type of knowledge and the manner they contribute to this target. The first method post-edits the current anaphora translations of SMT systems based on two scores, one comes from the gender and number of the antecedent of the target pronoun, and the other comes from the decoder’s search graph. Among all possible translations, the candidate with the highest global score, which is the linear combination of two above scores, will be chosen as the post-edition of the anaphora. The second method integrates a Coreference Model, which encodes (on a large training corpus) the probabilities of translating a source anaphora into the target counterpart given the gender and number of the target antecedent, into the SMT decoder. The new decoder uses its conventional phrase table every time it encounters all the other words than pronouns (“it” and “they”), and this new model with such words. The third method predicts the proper target translation for each source pronoun. The predictor is built from a set of morphological features, coming from the gender, number, person of preceding and following words of each source pronoun. It also takes into account the partial and absolute agreements on these attributes among such words. Show less
-
-
-
-
Lecturer
-
Dec 2008 - Sep 2011
I gave lectures for undergraduates and did research on Natural Language Processing I gave lectures for undergraduates and did research on Natural Language Processing
-
-
-
FPT University
-
Education Administration Programs
-
700 & Above Employee
-
Instructor
-
Sep 2007 - Sep 2008
I worked as instructor at FPT - Aptech Computer Education center. I was in charge of providing lectures and guiding projects on C#.NET modules. I worked as instructor at FPT - Aptech Computer Education center. I was in charge of providing lectures and guiding projects on C#.NET modules.
-
-
Education
-
Université Joseph Fourier (Grenoble I)
Doctor of Philosophy (Ph.D.), Computer Science -
Hanoi University of Technology
Master's degree, Computer Science -
Hanoi University of Technology
Bachelor of Engineering (B.E.), Information Technology
Community
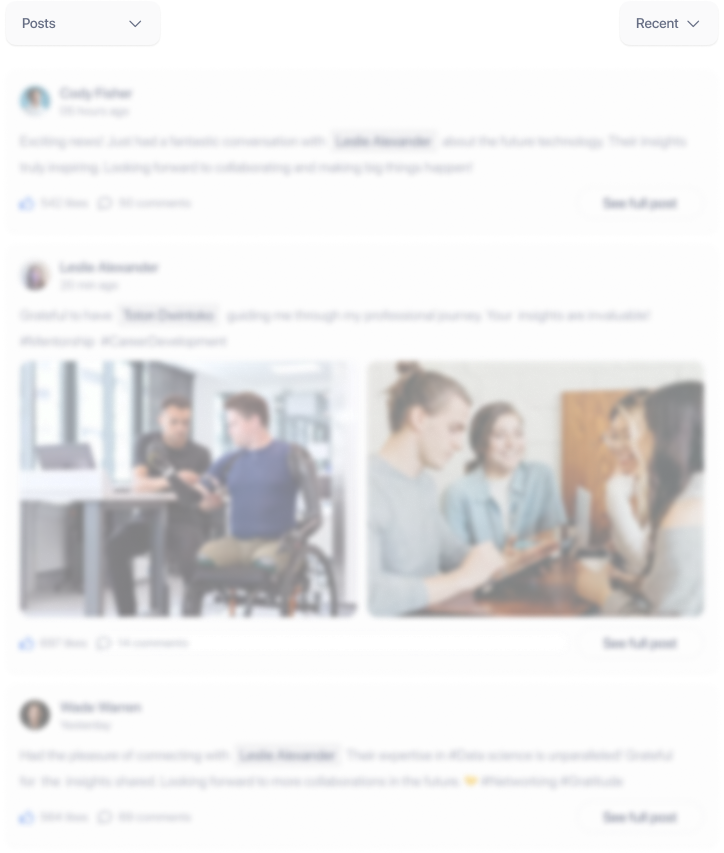